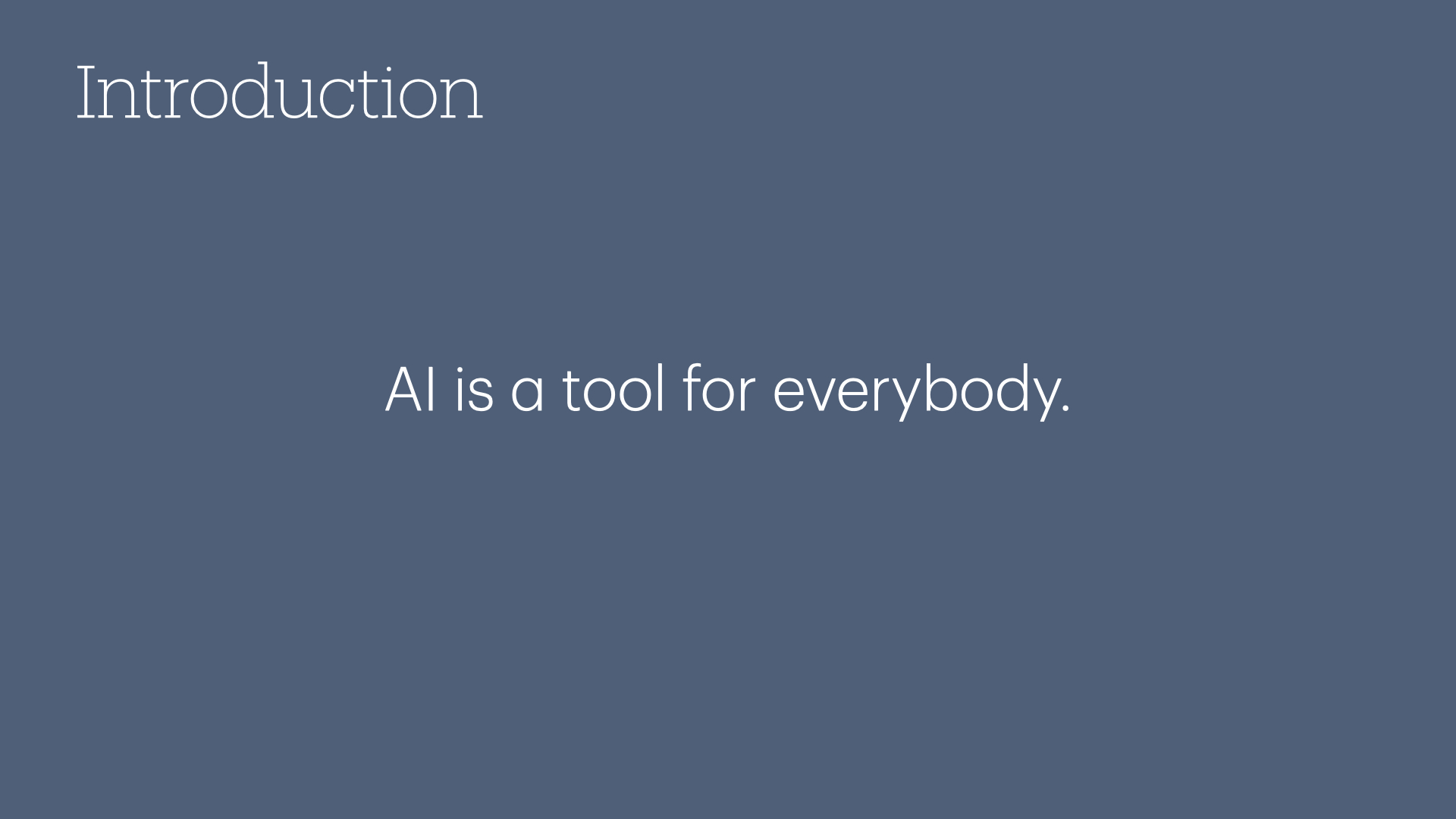
I firmly believe that AI is a tool for everyone.
Iâve been immersed in technology ever since I built my first website at eight years old. For the last three decades, I've eagerly followed every major technological breakthrough, examining each under the lens of "okay, so what's useful with this one?"
This recent breakthrough in AI technology, in particular, gives me the same level of excitement that I got when I built my first website or jailbroke my iPhone for the first time.
There is so much potential with AI, and the best part is that you don't need to know everything about AI in order to get value from itâjust a bit of training on how to integrate these tools into your life.
Think about your car: unless you're a gear head, you probably don't know the first thing about how pistons work within an engine, and yet you don't need to know that in order to drive it efficiently. You do, however, need take to take classes to learn how to operate it properly and safely.
The same goes for these new artificial intelligence tools. And here's some good news: like all of your ancestors before you, you can totally figure out how this new tool works with just a little guidance.
My hope is that this talk serves as the first step in your training process for learning about AI. You should leave here with a basic understanding of how these tools are designed to work, as well as some ideas for how to incorporate them into your life.
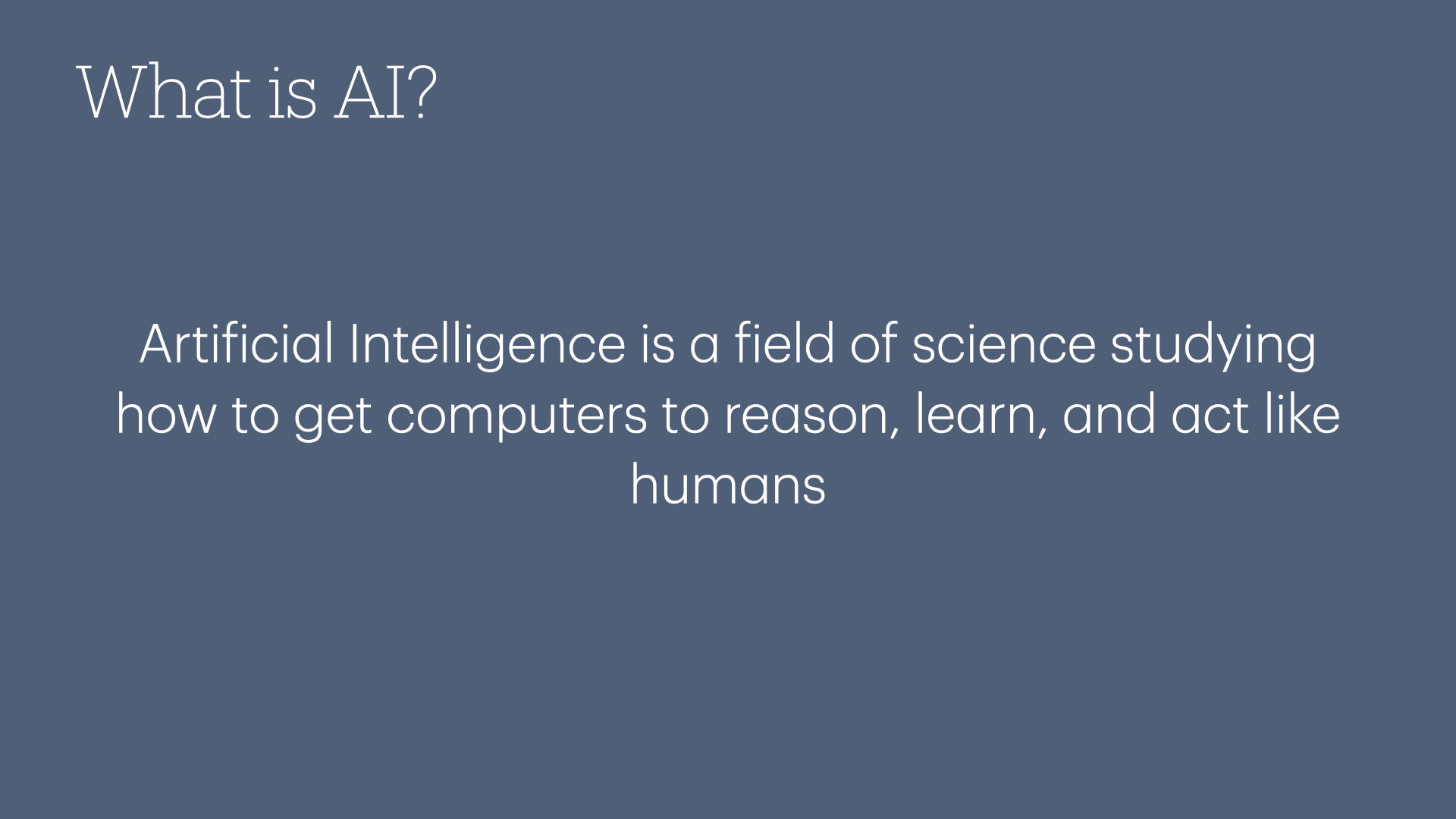
So, what is artificial intelligence?
Artificial intelligence is a field of science focused on getting computers to act, think, and reason like humans.
Human intelligence, unlike other forms we see in nature, excels at pattern recognition and decision-makingâtwo complex skills that AI aims to replicate.
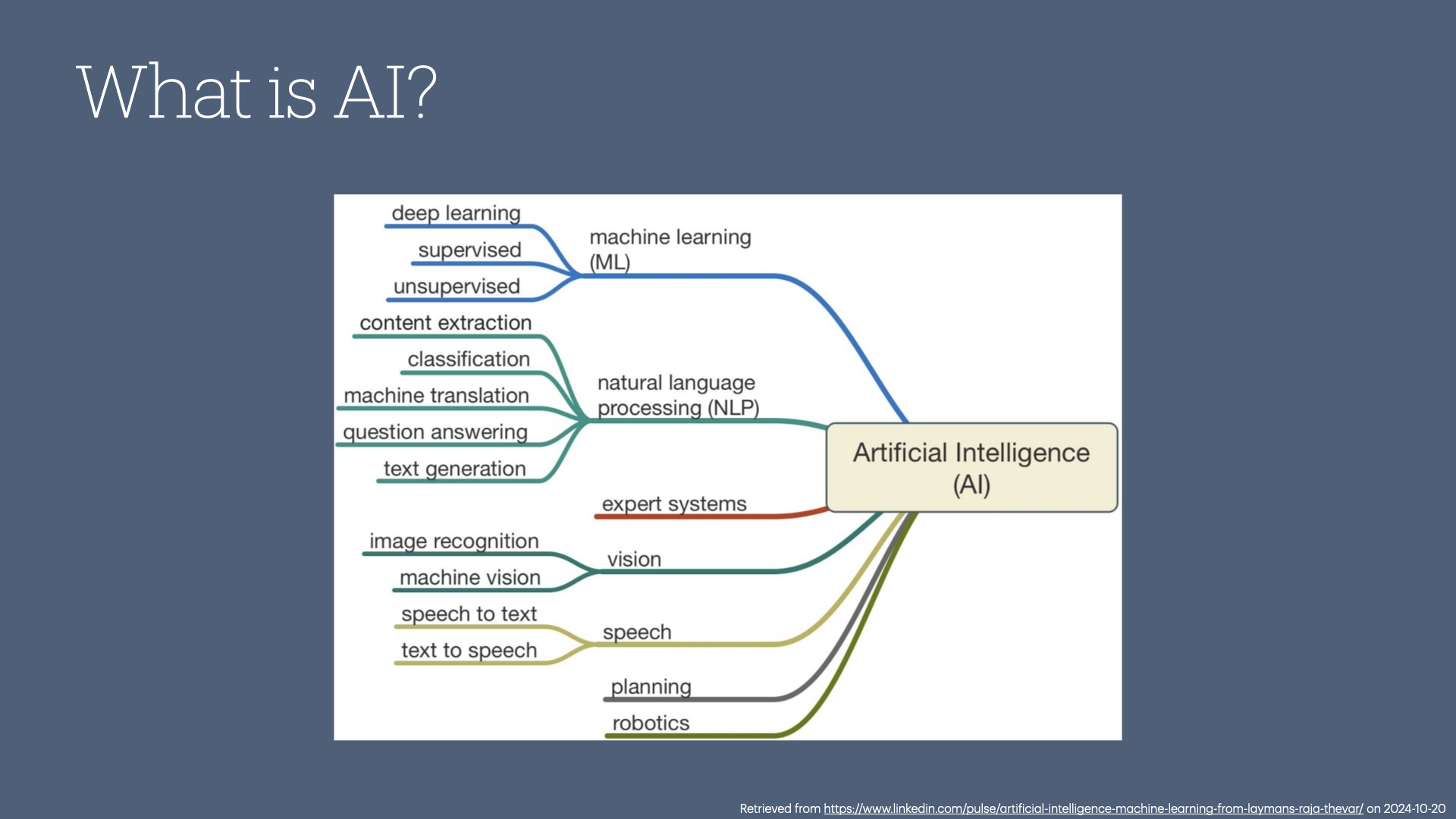
A common misconception about artificial intelligence is that it's one thing. While there are some who are working on artificial general intelligence (like HAL-9000), most researchers in the AI space aren't working on building an all-purpose form of intelligence. Instead, they focus on digitizing specific areas of intelligence.
For instance, natural language processing helps computers understand not just words but the meaning behind them, while computer vision enables machines to recognize and process visual information.
Each of these offshoots serves unique functions.
A helpful analogy is to think of AI as a toolkit, like walking into a hardware store and asking for a hammer.
The clerk will likely ask which kind because there are various typesâsledgehammers, jackhammers, ball-peen hammers, etc.
AI is similar; you need to know what problem youâre solving in order to choose the right tool.
Recently, advancements in AI have led to generative AI models, like ChatGPT and Googleâs Gemini, which can create new content. But to understand where generative AI fits, letâs discuss some foundational AI concepts.
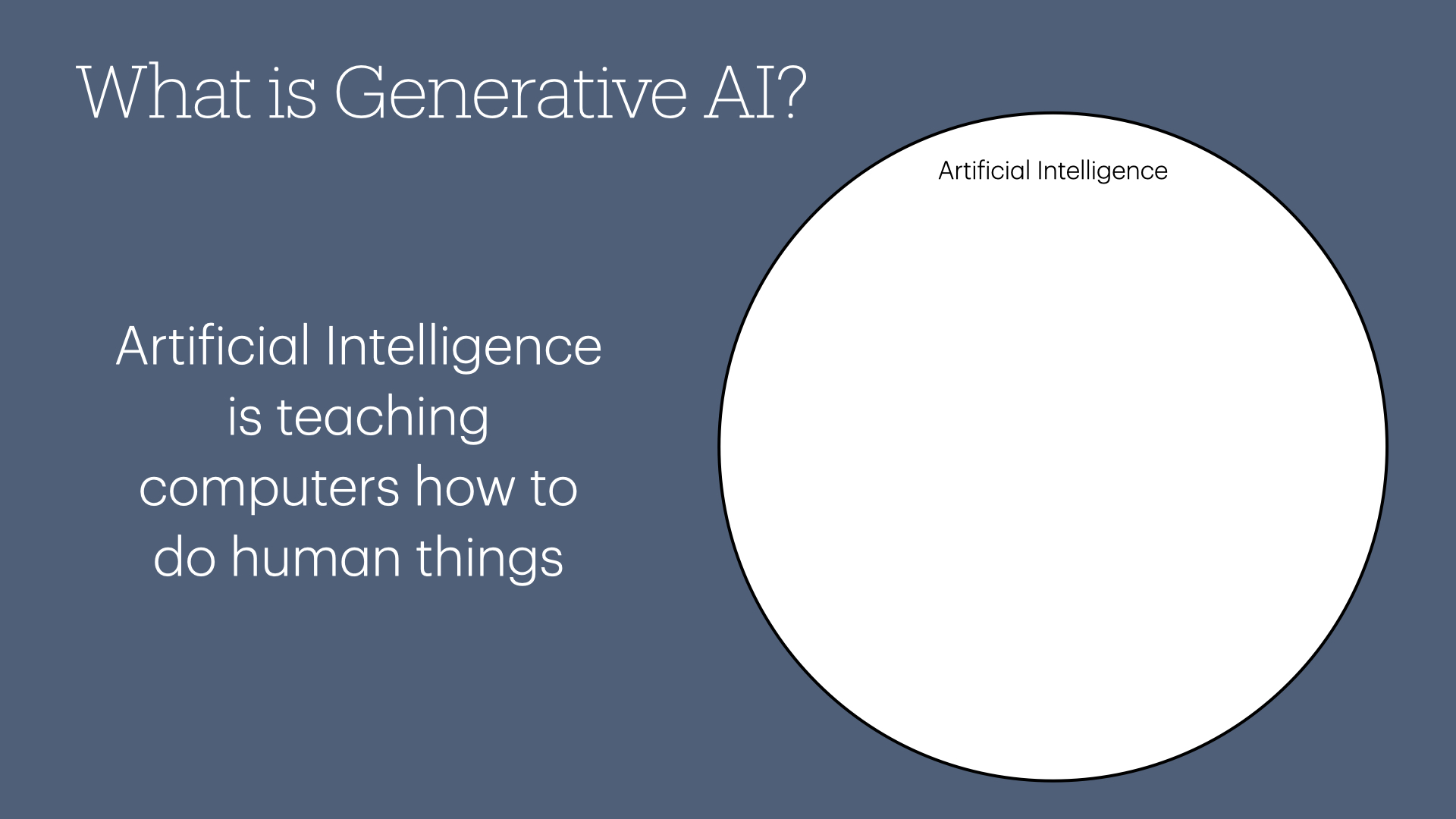
Artificial intelligence, as we discussed earlier, is a broad field focused on teaching computers to perform human-like tasks.
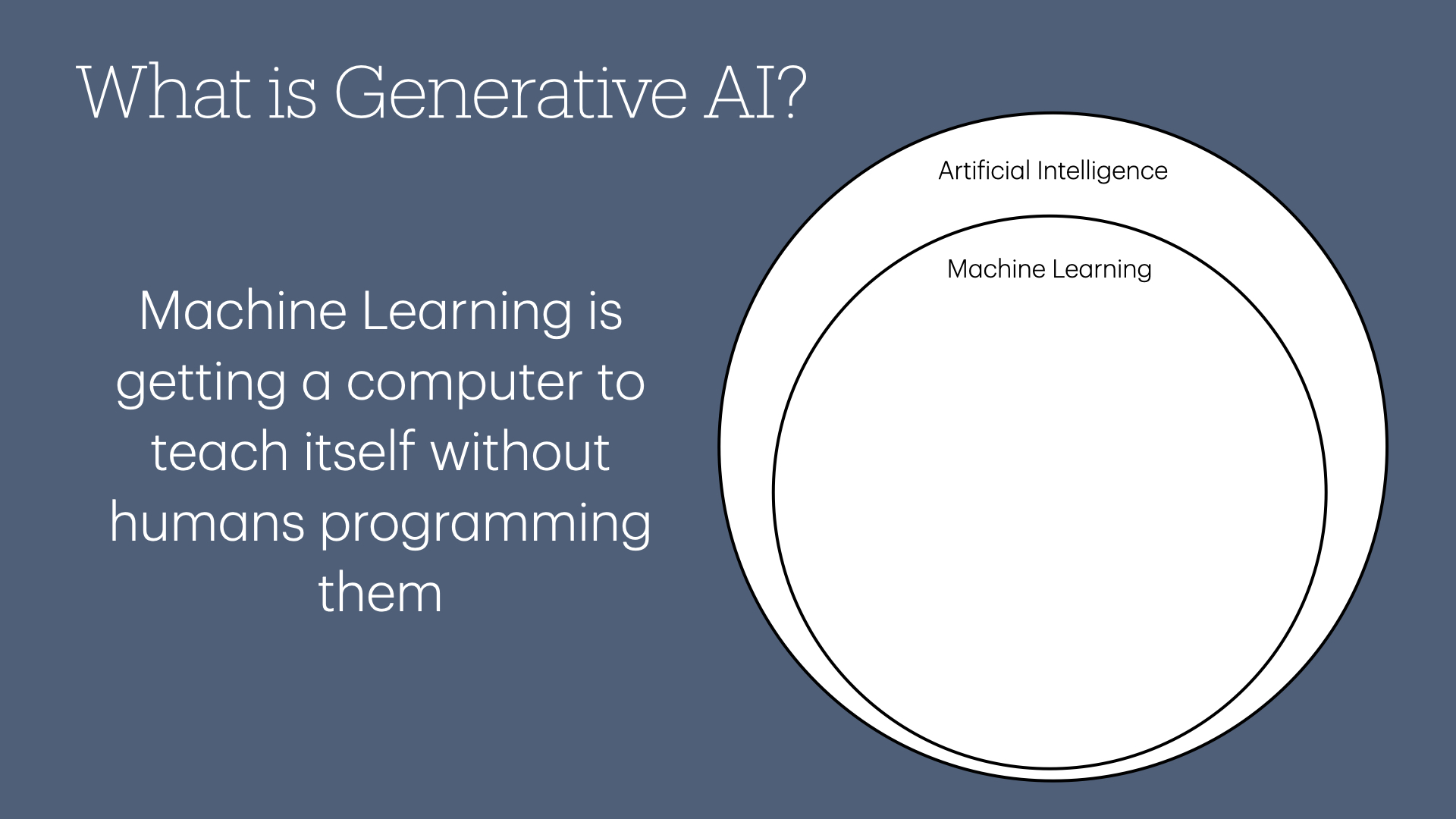
Within the broad field of artificial intelligence, there's machine learning, where we teach computers to learn without direct human programming.
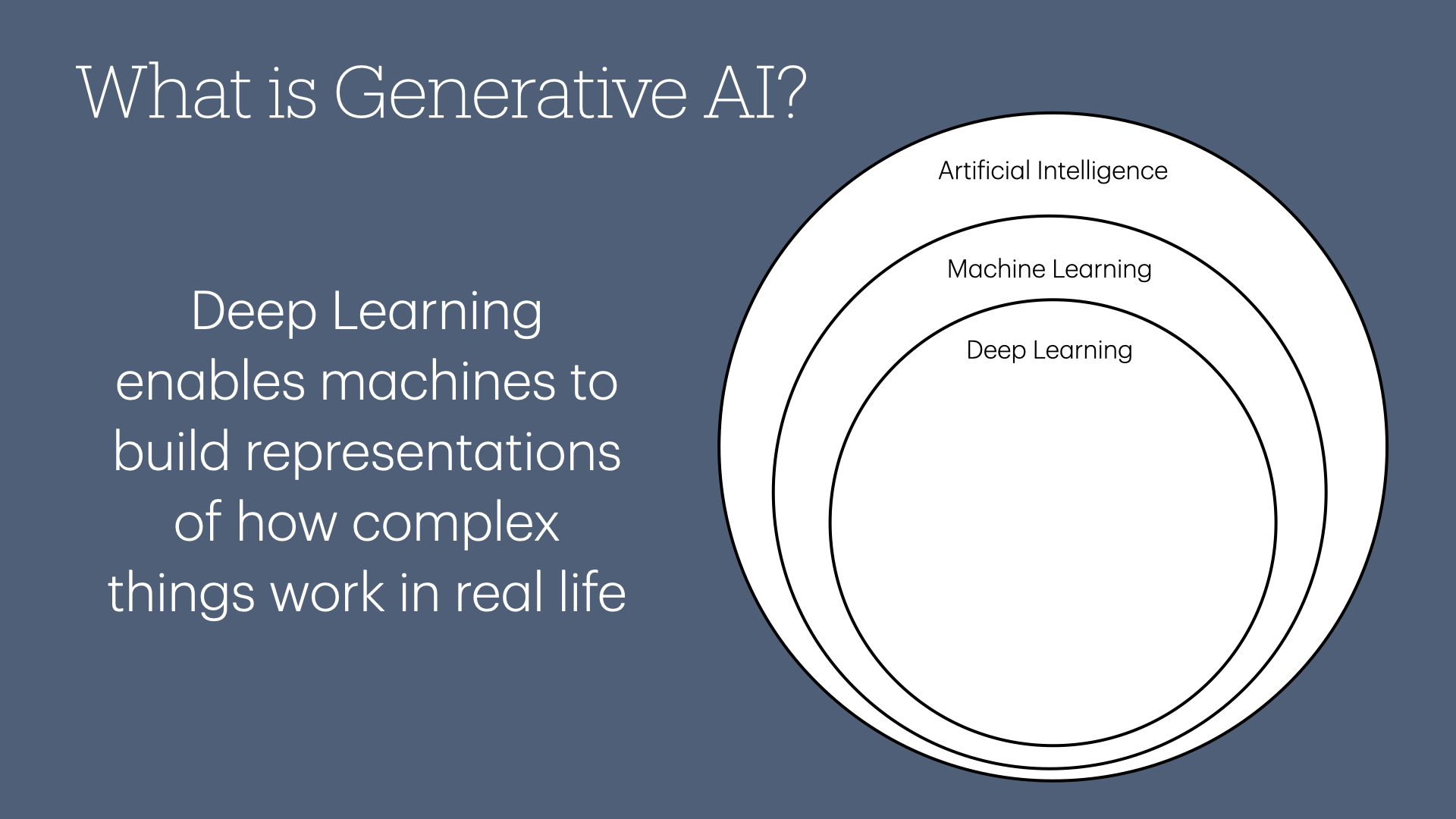
A subset of machine learning is deep learning, which allows computers to create complex digital representations of real-world objects.
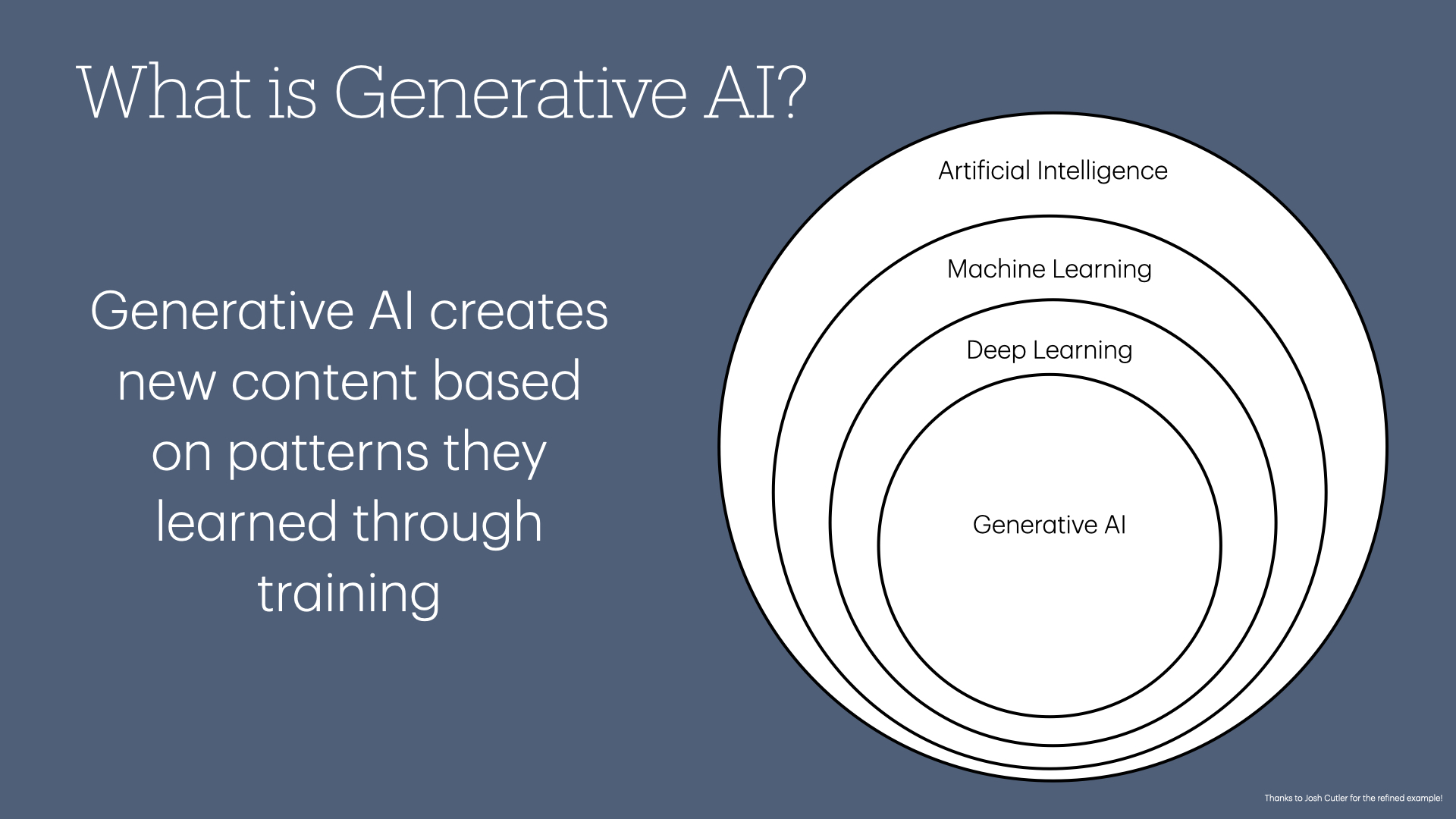
After reaching this level, we enter generative AI, where computers use learned representations to generate new content based on recognized patterns.
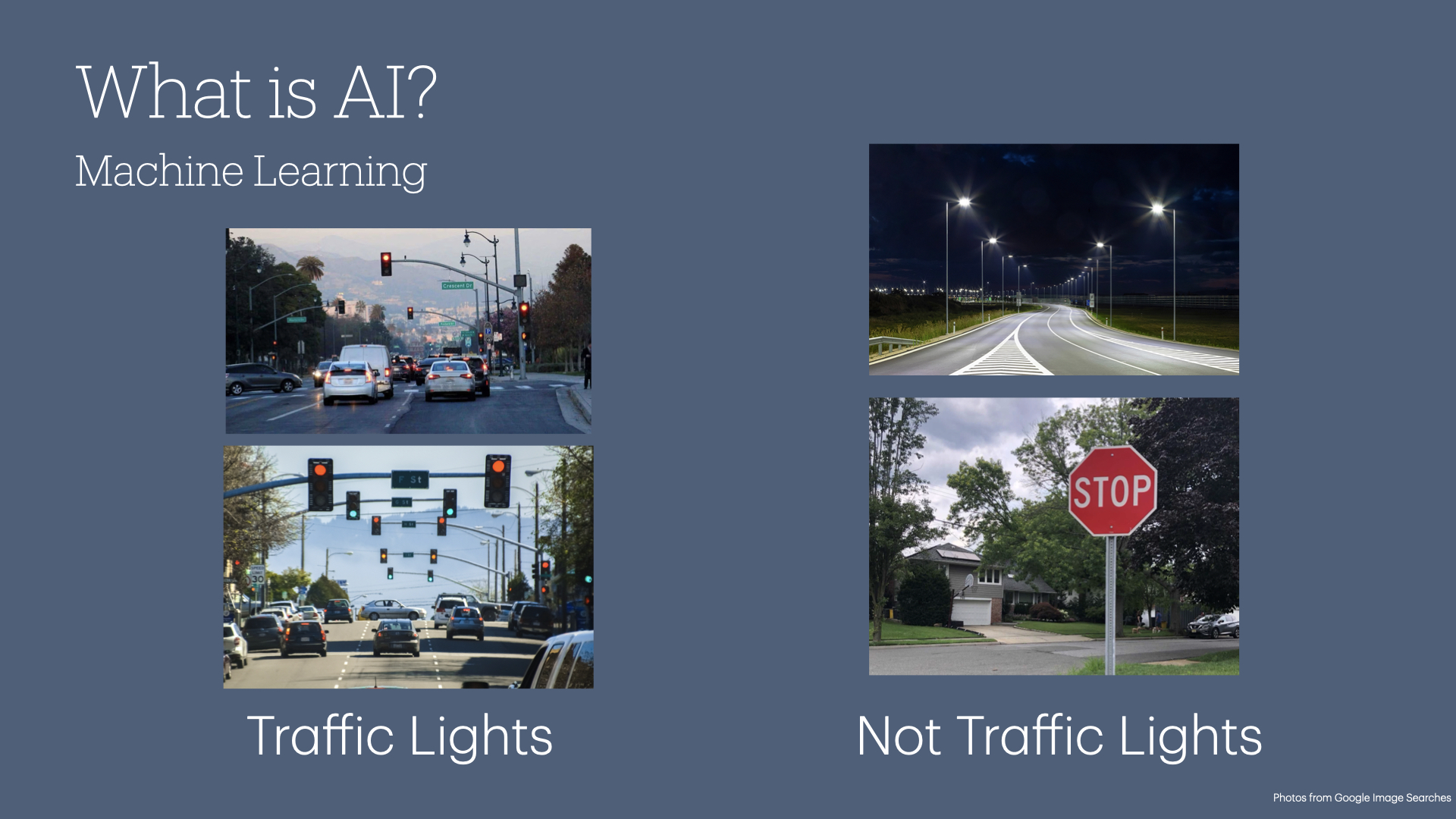
To explain machine learning, imagine teaching a computer to recognize a traffic light.
Youâd feed it thousands of pictures of traffic lights and train it to differentiate between traffic and non-traffic lights.
After undergoing thousands (or even millions) of tests, the computer program can predict with increasing accuracy, for example, âYes, this is a traffic light,â or âNo, this is not a traffic light.â
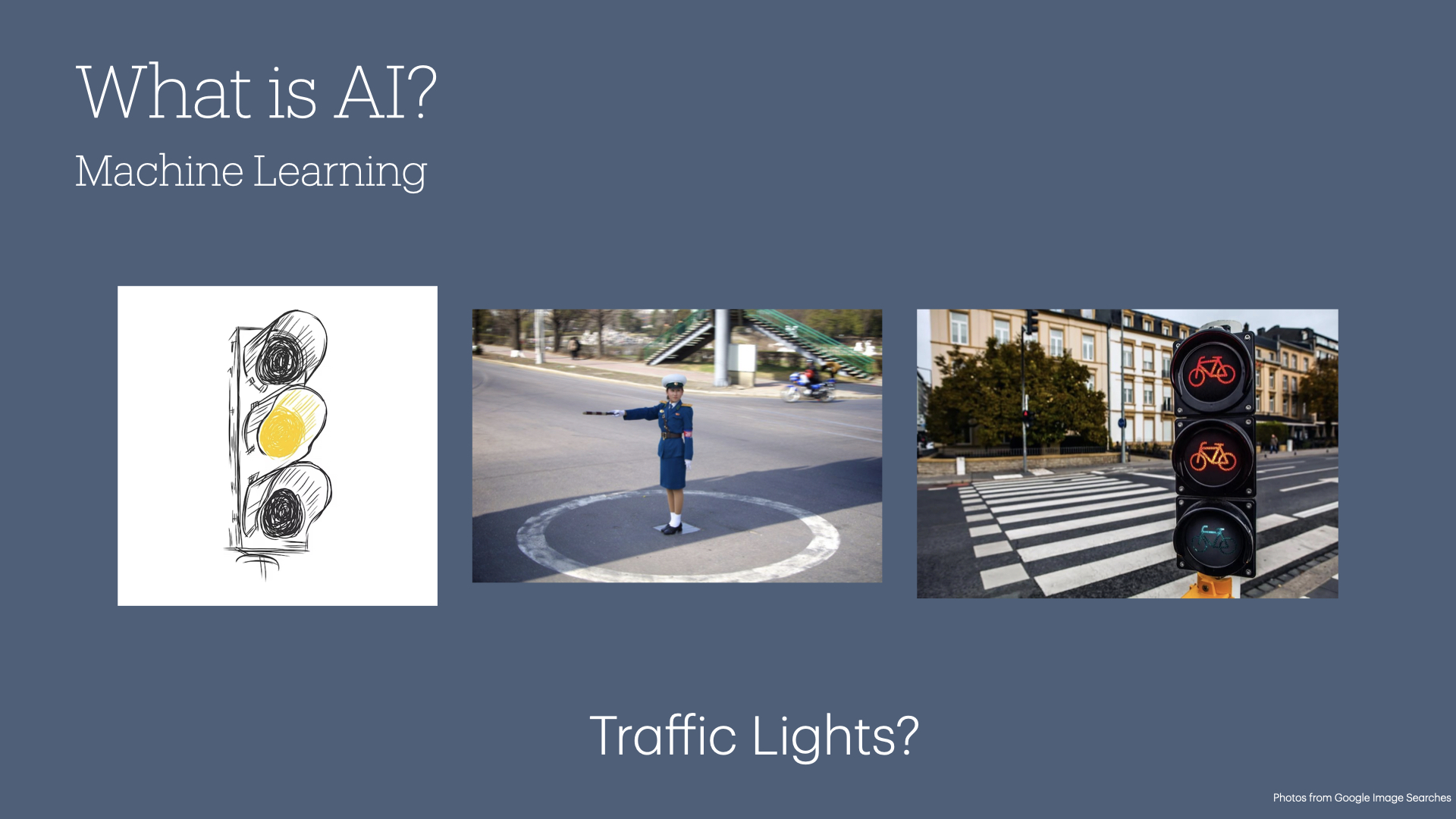
You want to make sure during its training that you give it data relevant to the task you want it to perform.
For example, edge cases arise.
- Do you want your model to say that a hand-drawn traffic light counts as a traffic light?
- Some countries don't use traffic lights, but rather use humans to direct traffic... do those count?
- Newer traffic lights are geared toward specific modes of transportation, like bicycles. Are those traffic lights?
As you make these decisions and label your data accordingly, the training process leads to a model capable of identifying traffic lights based on patterns itâs learned.
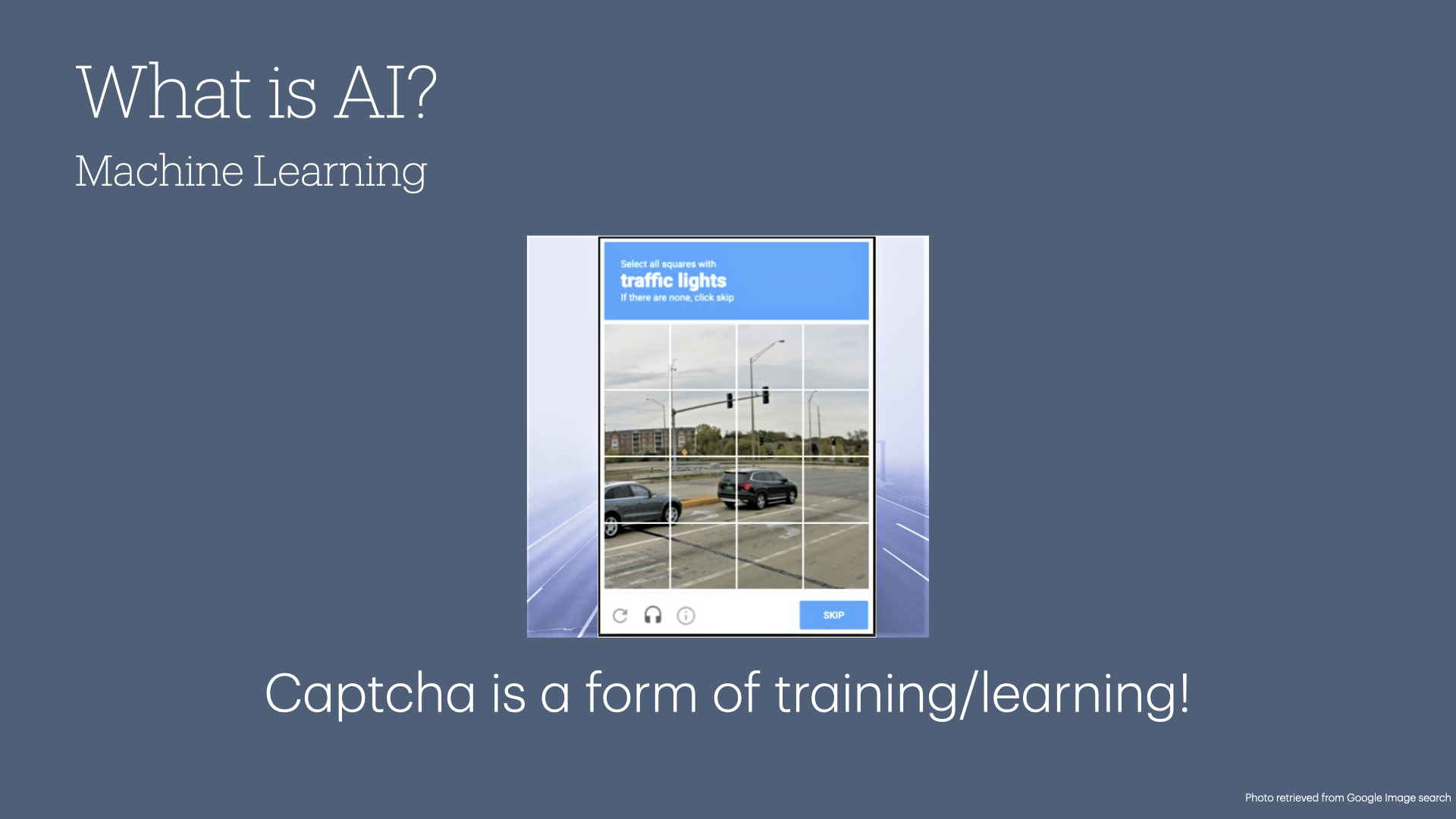
(By the way: every time you fill out a Captcha online, you are helping Google to train its models to recognize various elements it may encounter on the road. Thanks for the free labor, everyone!)
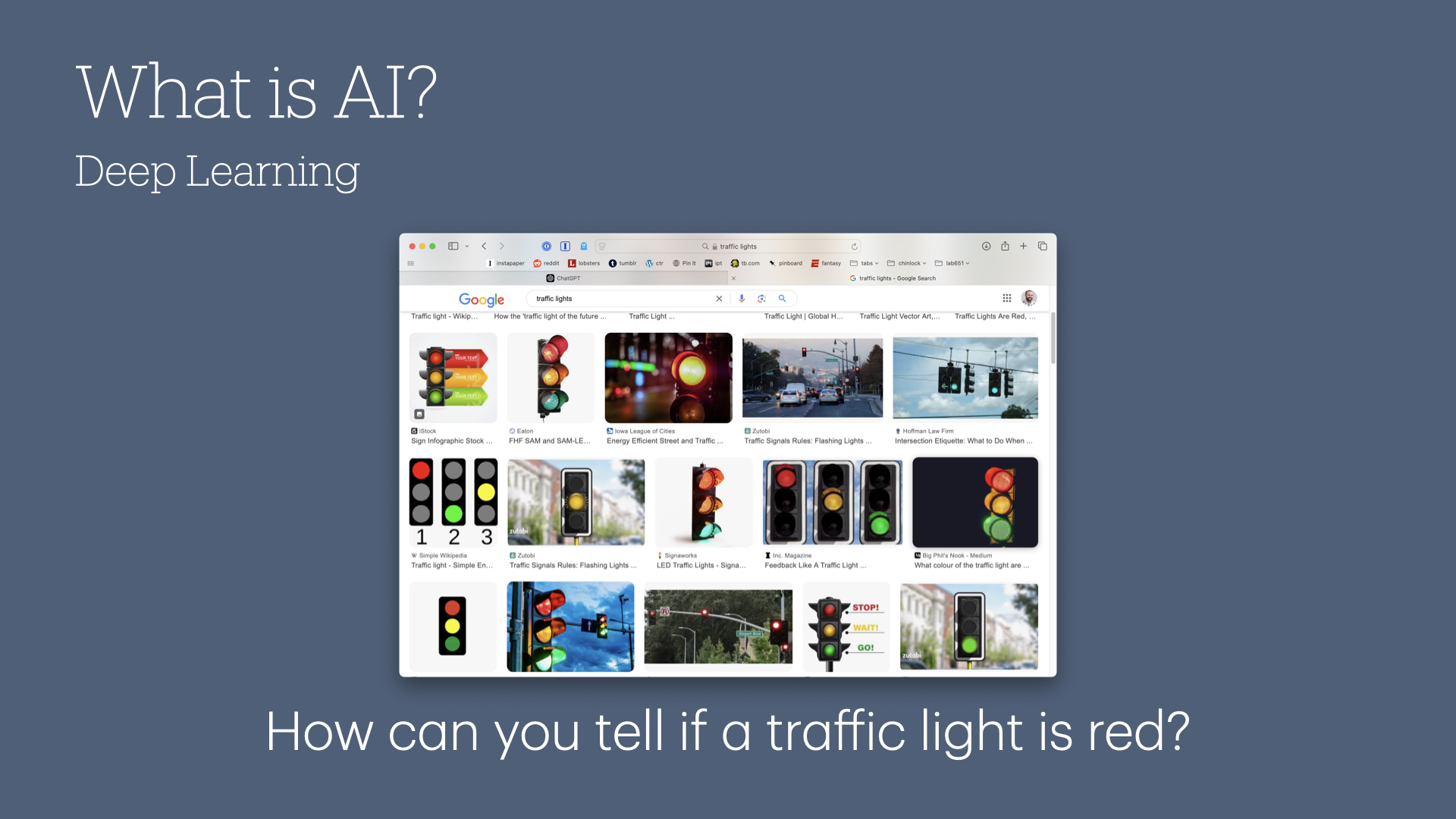
Machine learning is cool and has a ton of practical use cases, but what if we wanted to have the computer understand something more complex, like the color of the traffic light?
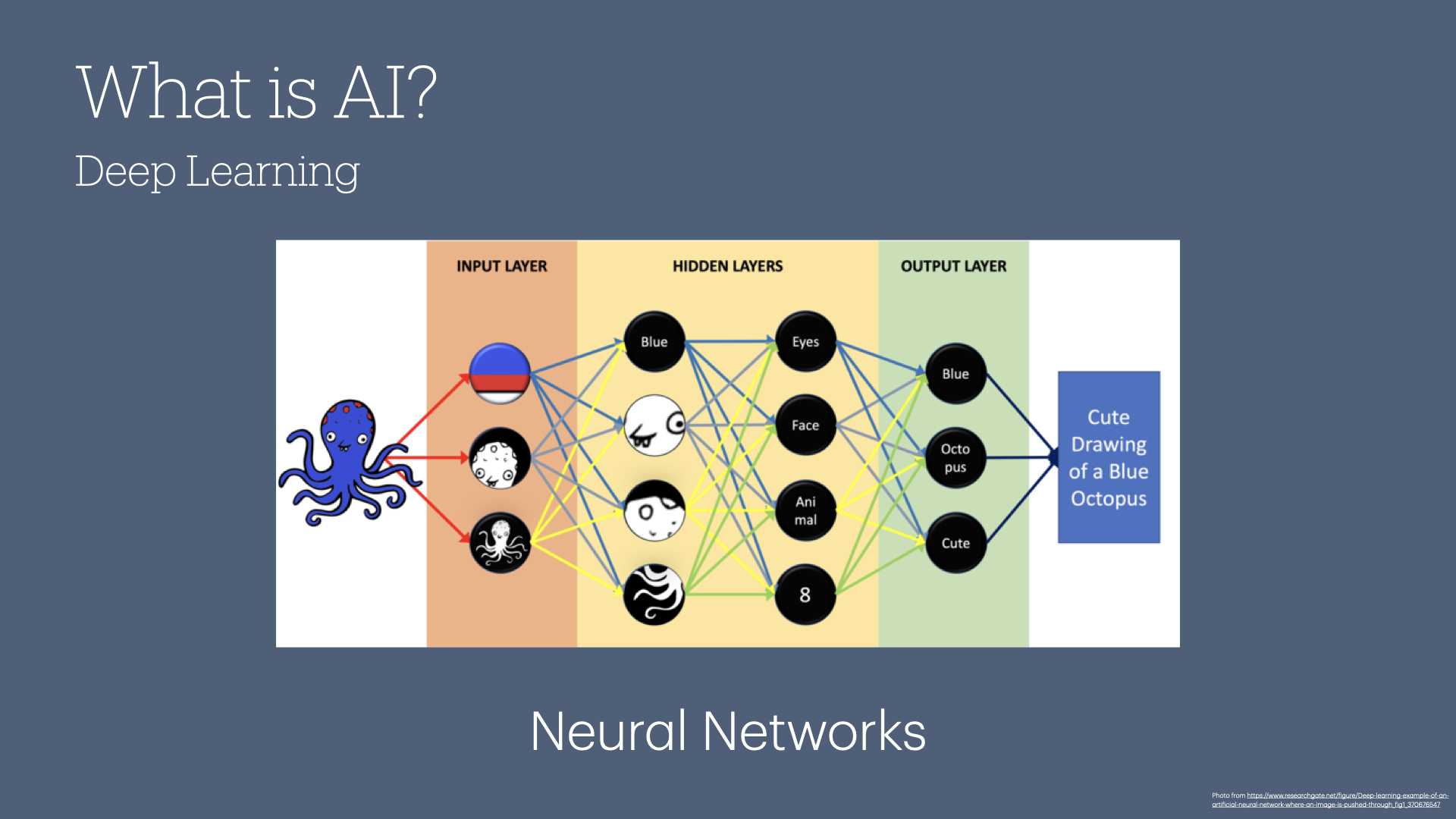
Deep learning takes machine learning a step further, using neural networks to analyze data in stages, like a detective reconstructing a crime scene. At each stage, the network gathers specific detailsâcolors, shapes, texturesâand then combines these details into a fuller, more nuanced picture, like a detective piecing together a mystery from small clues.
With our traffic light example, each layer in our neural network focuses on specific aspects of the image, such as color, shapes, or textures, to interpret complex visuals, like recognizing whether a traffic light is red, yellow, or green.
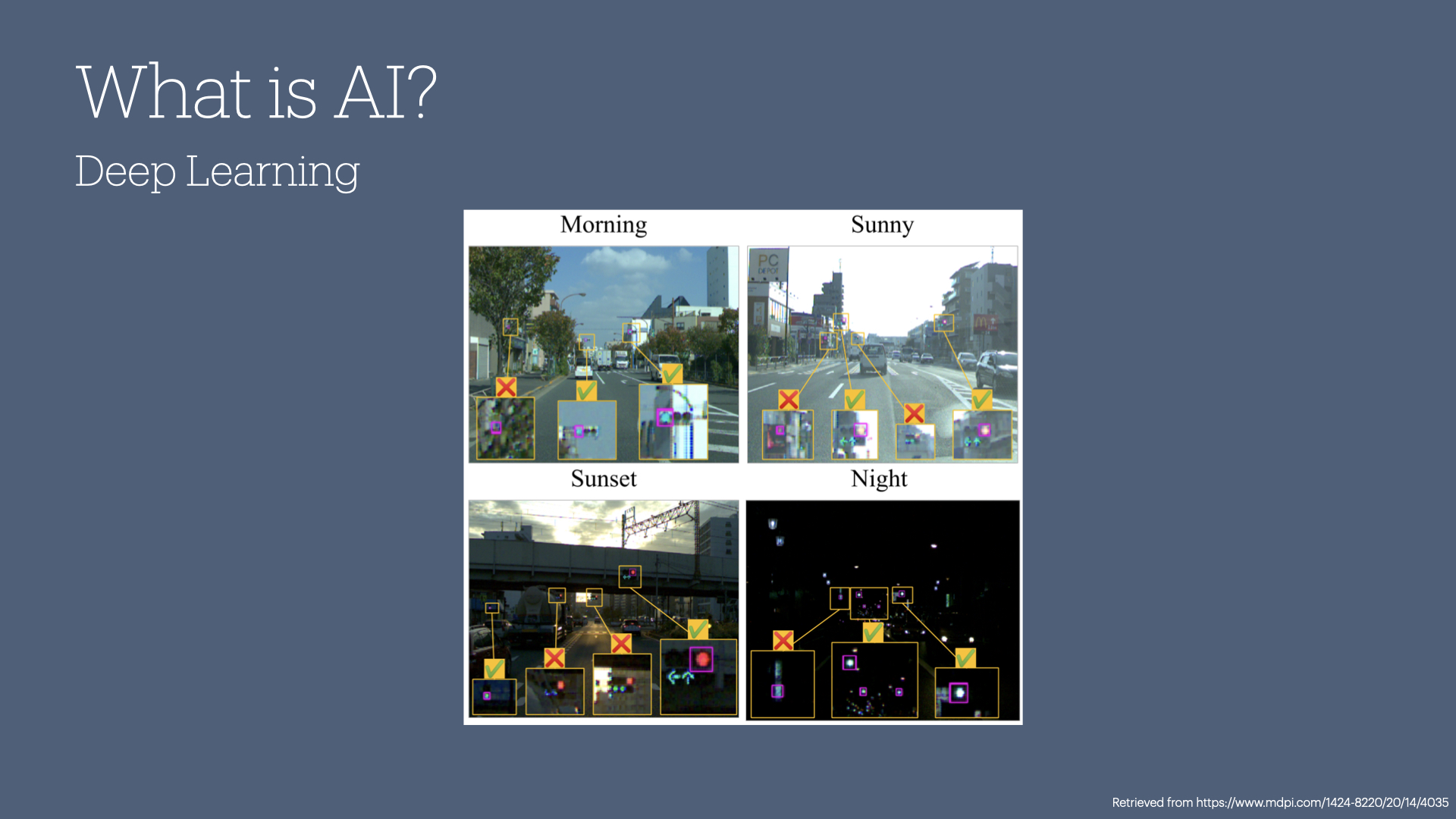
This depth is essential, especially in dynamic environments like self-driving cars, where traffic lights look different depending on the time of day, weather conditions, or lighting.
With enough examples, deep learning models can accurately identify traffic lights in all these conditions, forming the backbone of many AI applications, including autonomous vehicles and medical imaging.
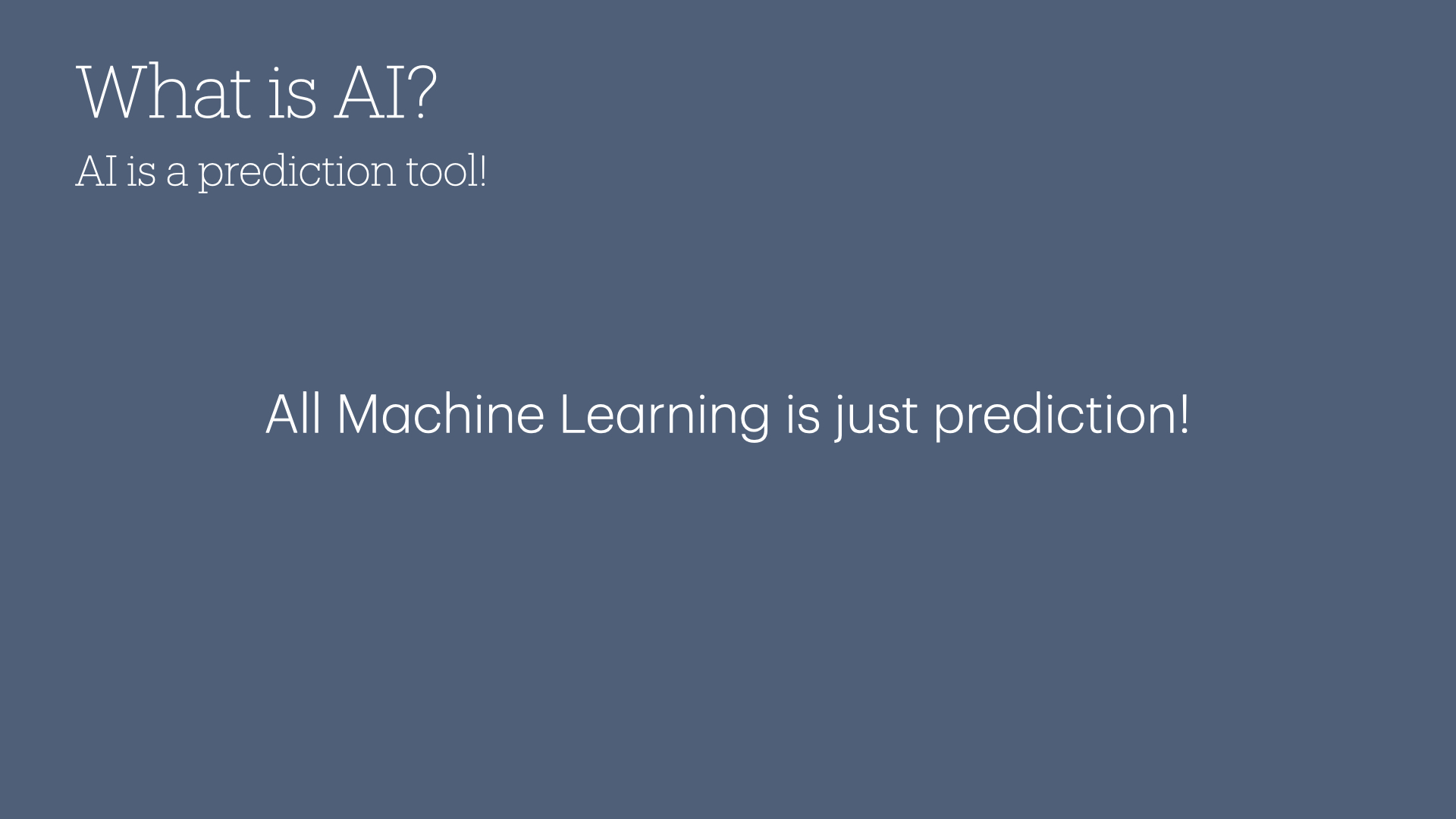
The big takeaway about machine learning and deep learning is that they're primarily tools for making well-optimized predictions based on patterns in past data. They use advanced probability and optimization to make 'best-guess' predictionsâcalculations that may seem insightful but are based purely on mathematical patterns, not true understanding.
None of this stuff is actually "alive" or "conscious" (as best we can tell... more on that in the "black box problem" section below).
All it is doing is saying "based on what I've learned while training on the data you gave me, I am making a prediction that this image contains a traffic light, or this image contains a "green" traffic light."
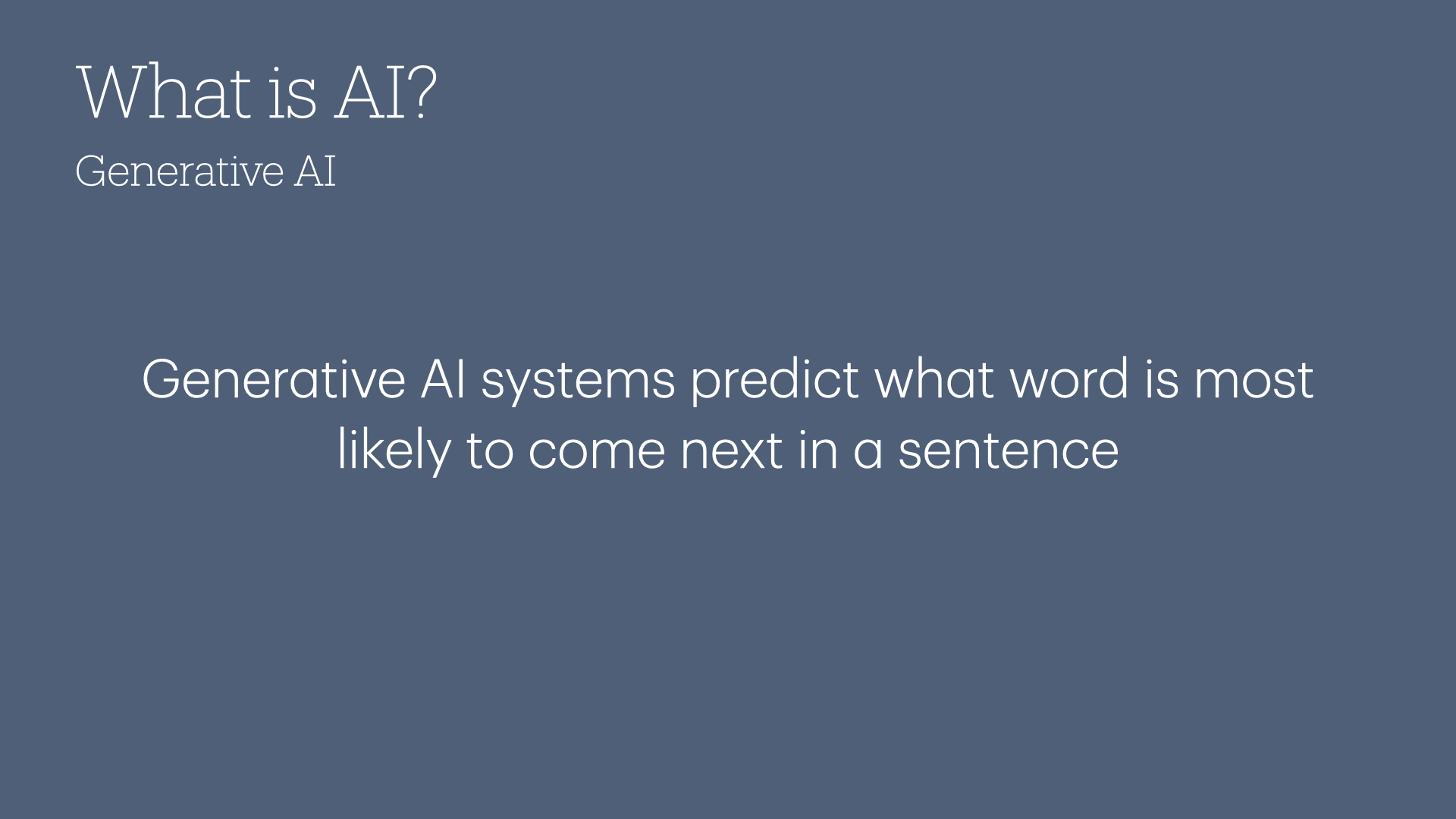
Now, letâs take it further.
What if instead of guessing what is inside an image, we can take these models and have them predict what what word comes next in a sentence?
That's what generative AI is doing!
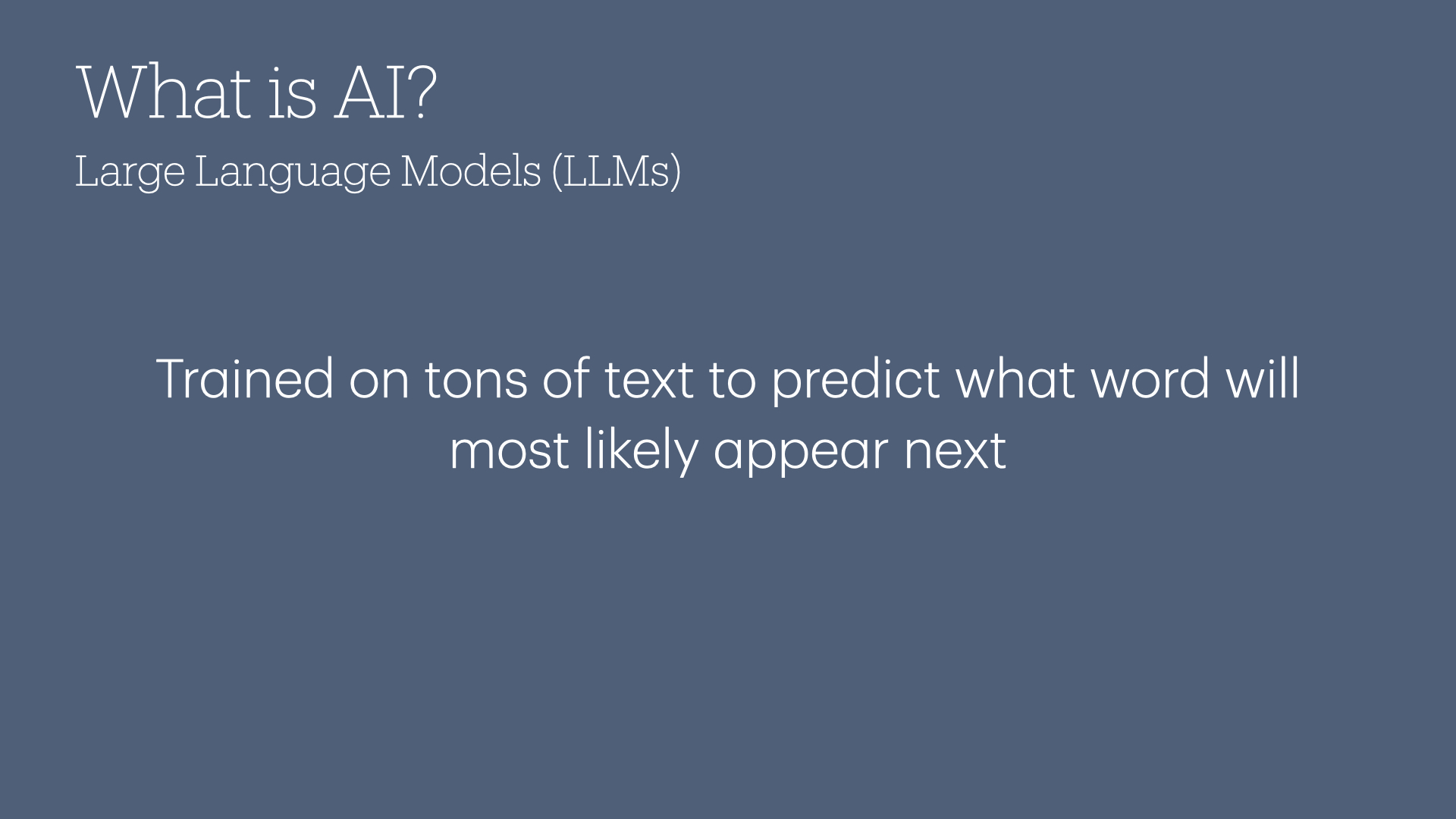
By training a neural network on vast amounts of textâlike public domain books, Reddit comments, and YouTube transcriptsâthe model becomes exceptionally skilled at predicting the next word in a sentence, mimicking human-like responses across countless topics.
And that's what a large language model does!
If you give a prompt to one of these systems, it will use all the patterns it recognized in training and spit out a very convincing answer to your prompt.
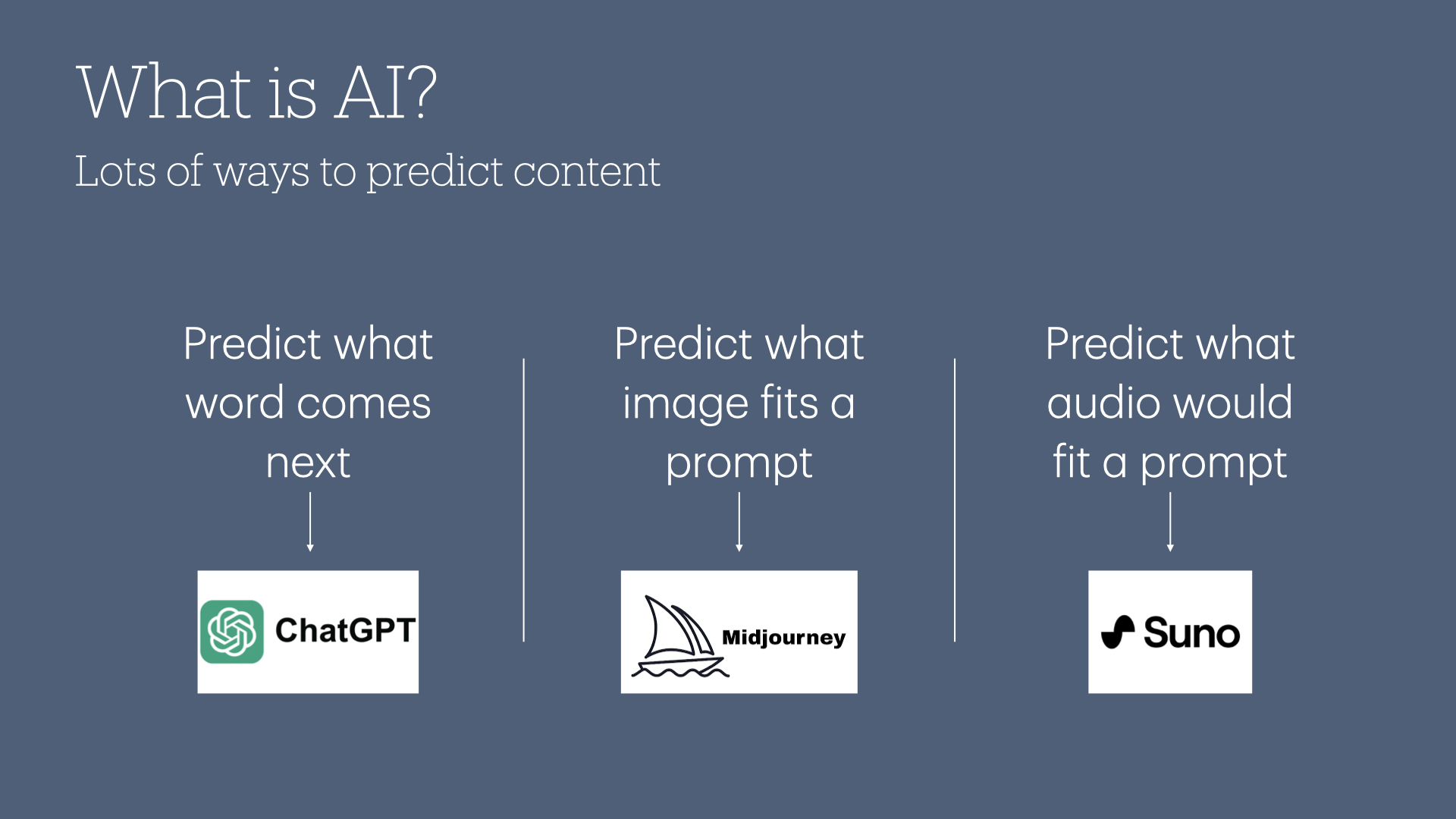
And even more impressive: you can run these models across all kinds of mediums.
Because under the hood, all generative AI tools (ALL of them!) are just running statistical predictions to guess at what is the most likely thing to happen.
If you want a model that can predict what word would come next in a sentence, you'd use ChatGPT or Gemini or Claude.
Images? Midjourney, DALL-E, etc.
Music? Suno.
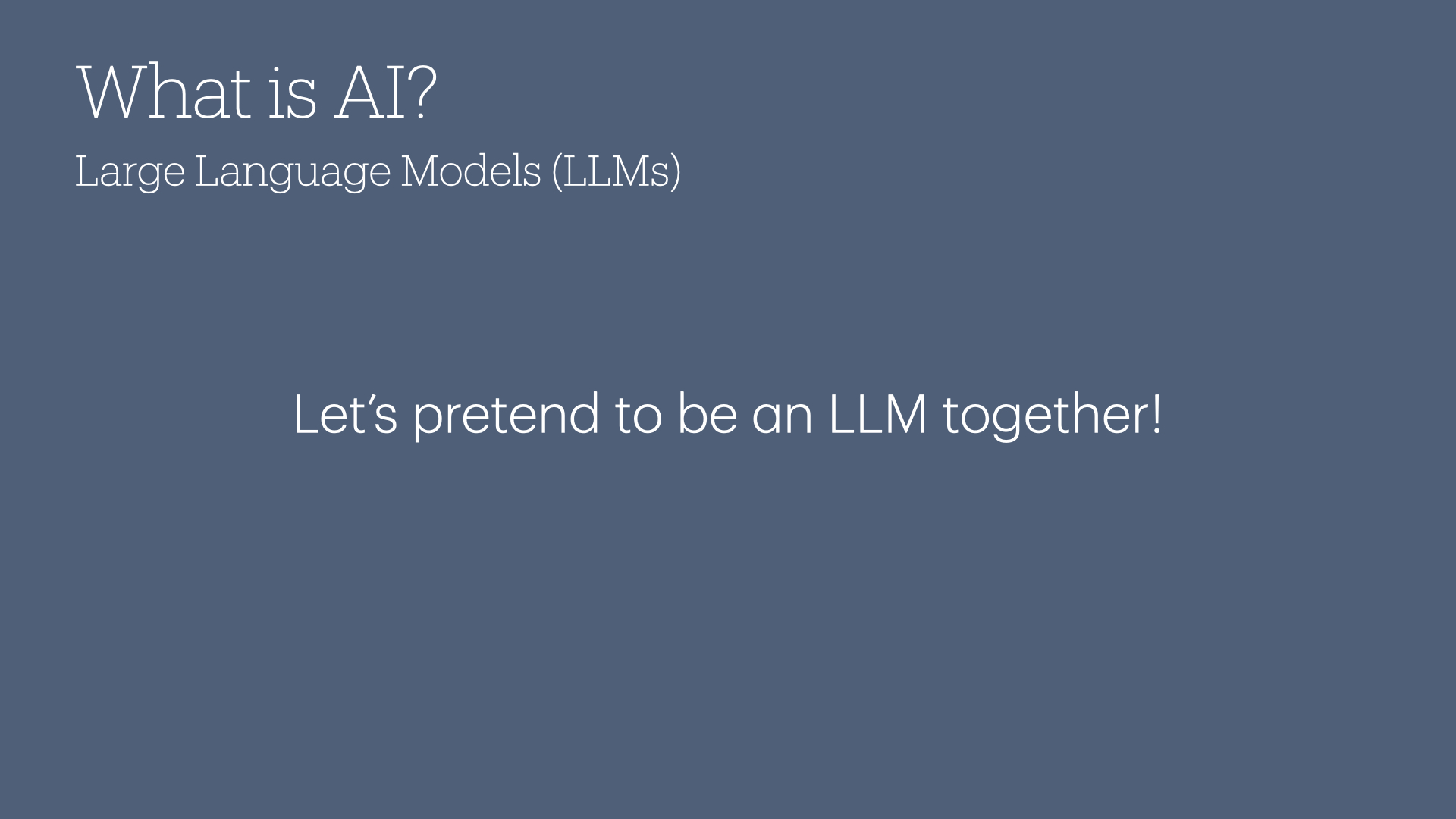
At this point, I imagine you are either thinking I'm talking about witchcraft, magic, or complete gibberish... and I suppose at some level, each of those is possible.
But stick with me here while I drive home this point about how these prediction systems work by having the audience here be my collective large language model.
So I'll give you a prompt, and I want you to fill in the blank:
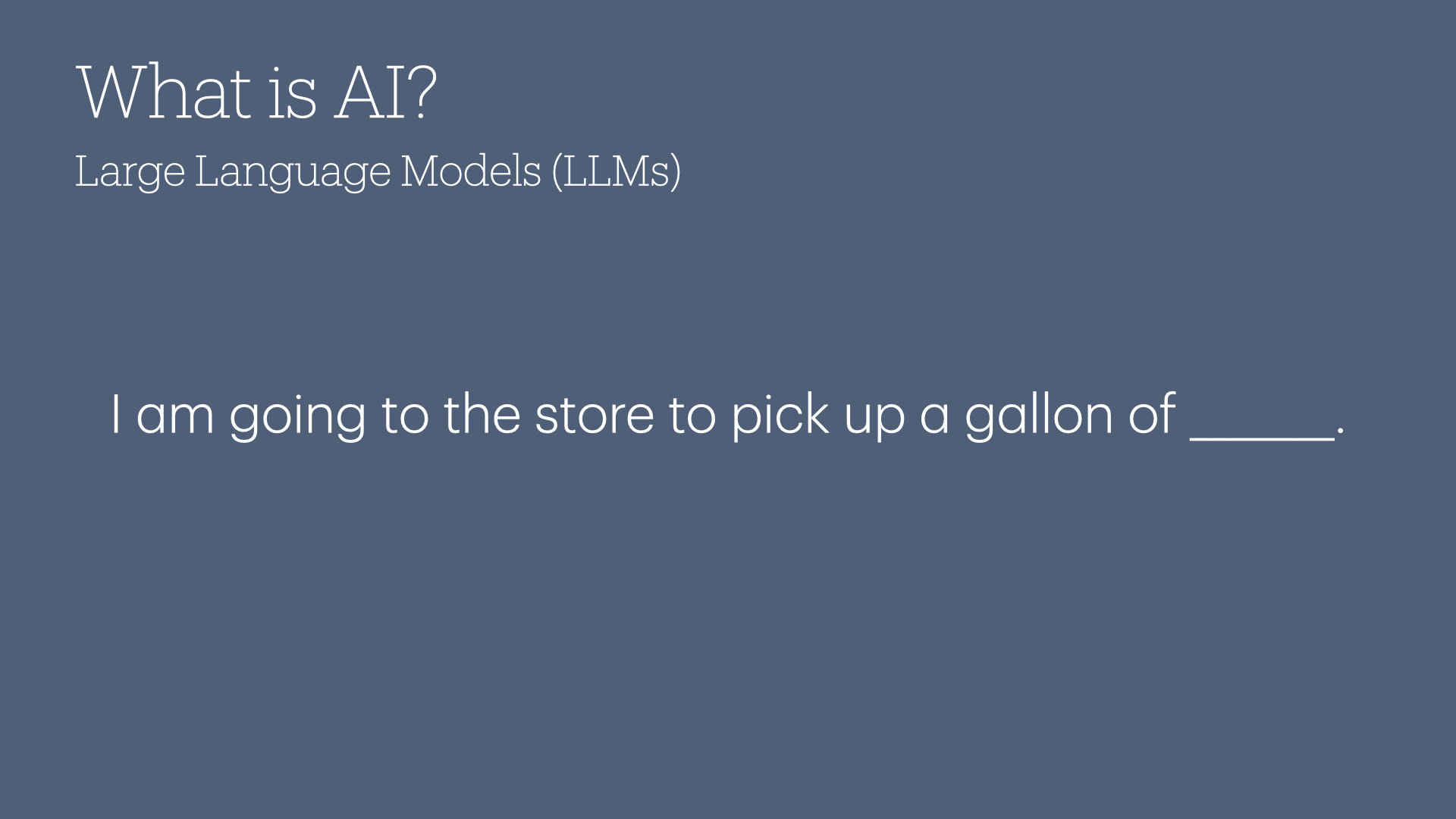
If I ask you "I am going to the store to pick up a gallon of ______", what would you likely fill that in with?
(In this case, the live audience of this webinar universally said "milk", but I've also heard people say "ice cream", and I can definitively say that those are my kind of people.)
There's one small problem though: I actually didn't get the answer I was looking for. đŹ
So I'm gonna give you a different prompt and see if I can get the answer I was looking for.
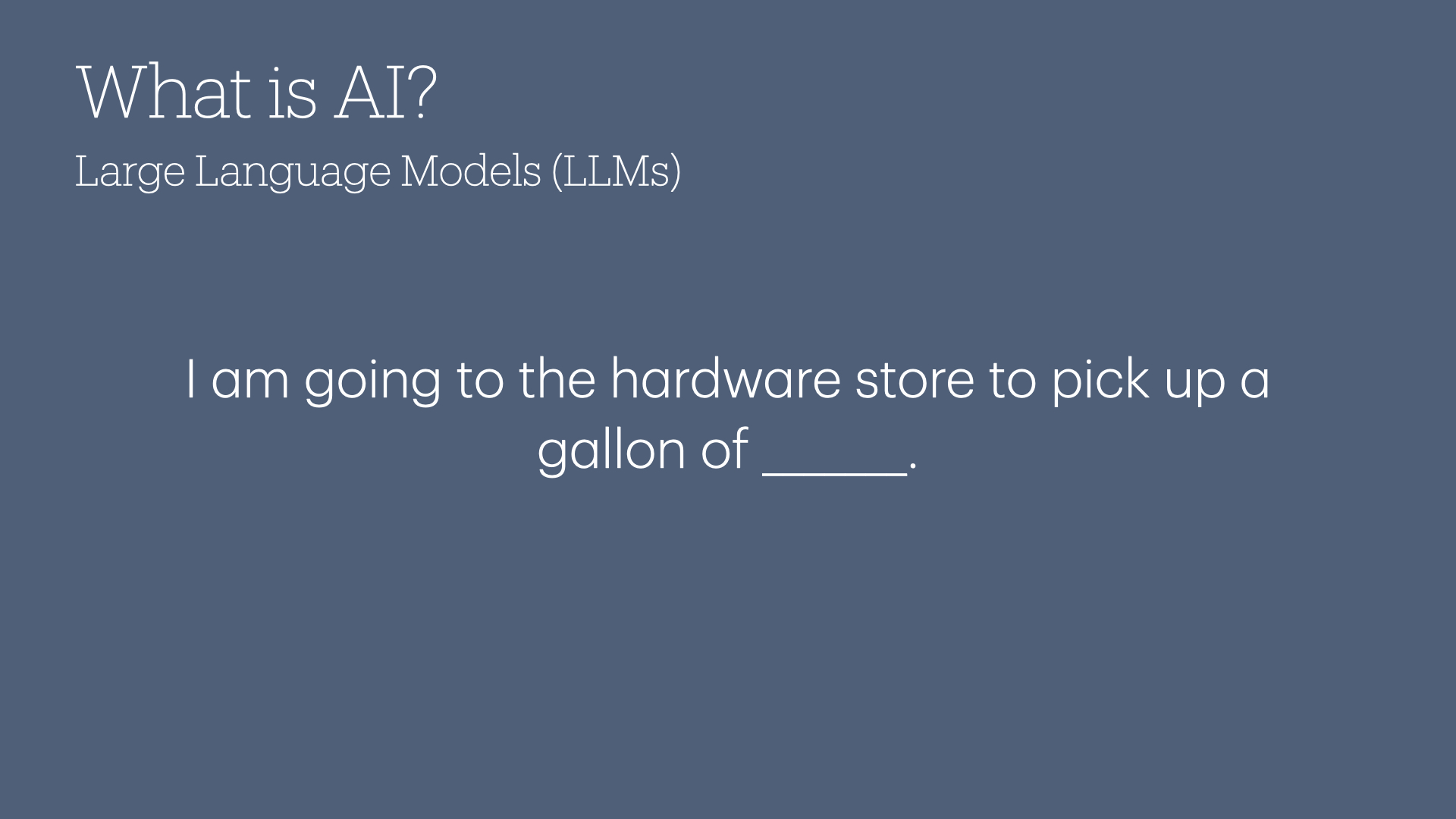
"I'm going to the hardware store to pick up a gallon of ______".
(In this case, the live audience universally said "paint", which was the word I was originally looking for.)
When you read the sentence for my first prompt and see "store", you subconsciously tap into your previous experiences with the word. If you grew up in Minnesota like me, you associate the word "store" with concepts like "grocery store", "Target", or "Walmart."
In that context, you are gonna be thinking about what they sell by the gallon in those places. Again, that's likely milk or ice cream.
In my second prompt, your brain is airlifted out of Target and dropped into a Menards or Home Depot. In this new context, you aren't thinking about milk anymore. You're thinking about paint, oil, water, or other chemicals that are sold by the gallon.
This shift in prompt context illustrates how generative AI works: it predicts based on the most likely answer, given the context.
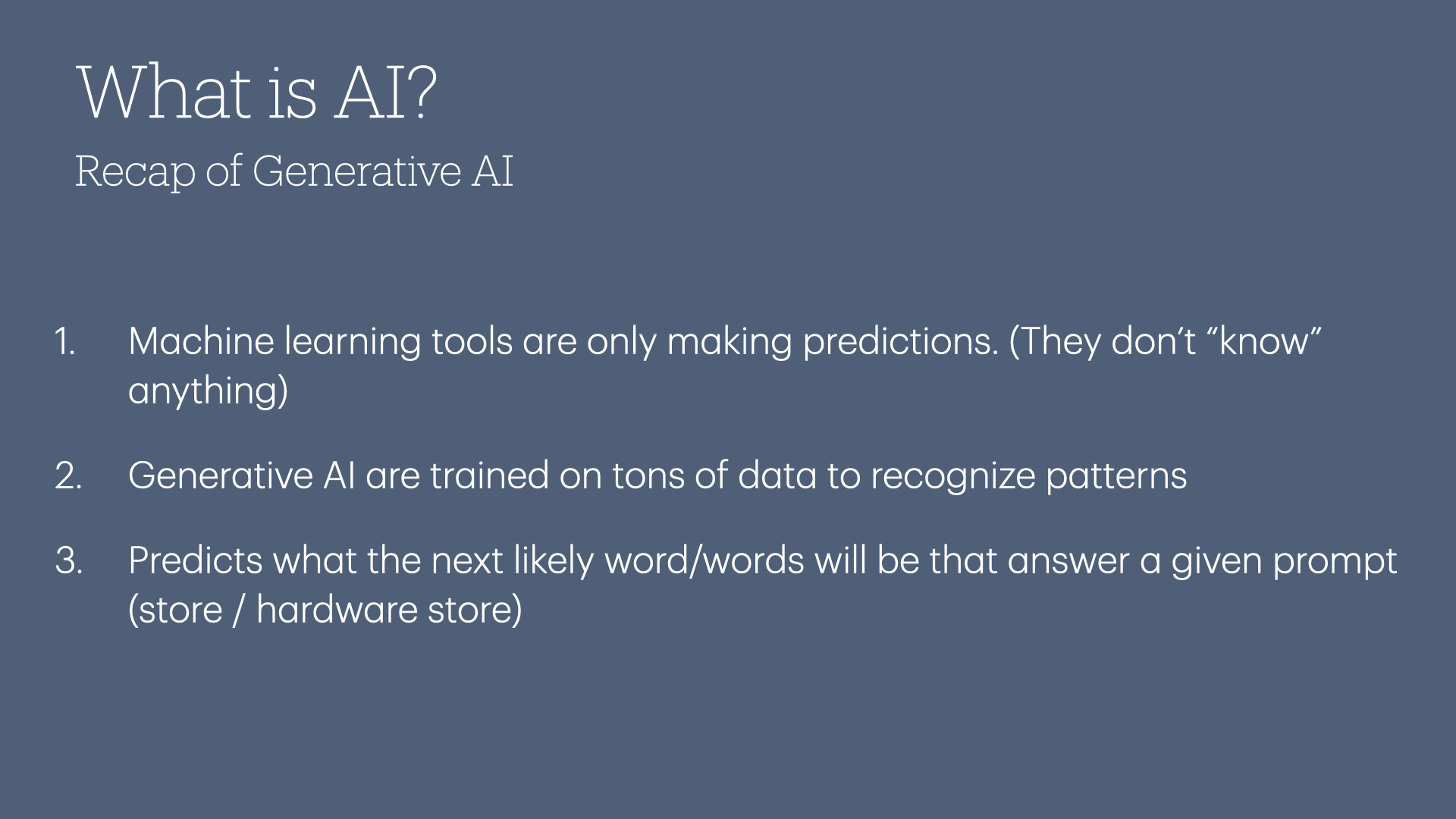
So, in summary: machine learning and deep learning models are about making predictions based on patterns in data.
Generative AI takes that one step further, creating new content based on whatâs likely to come next in a sequence.
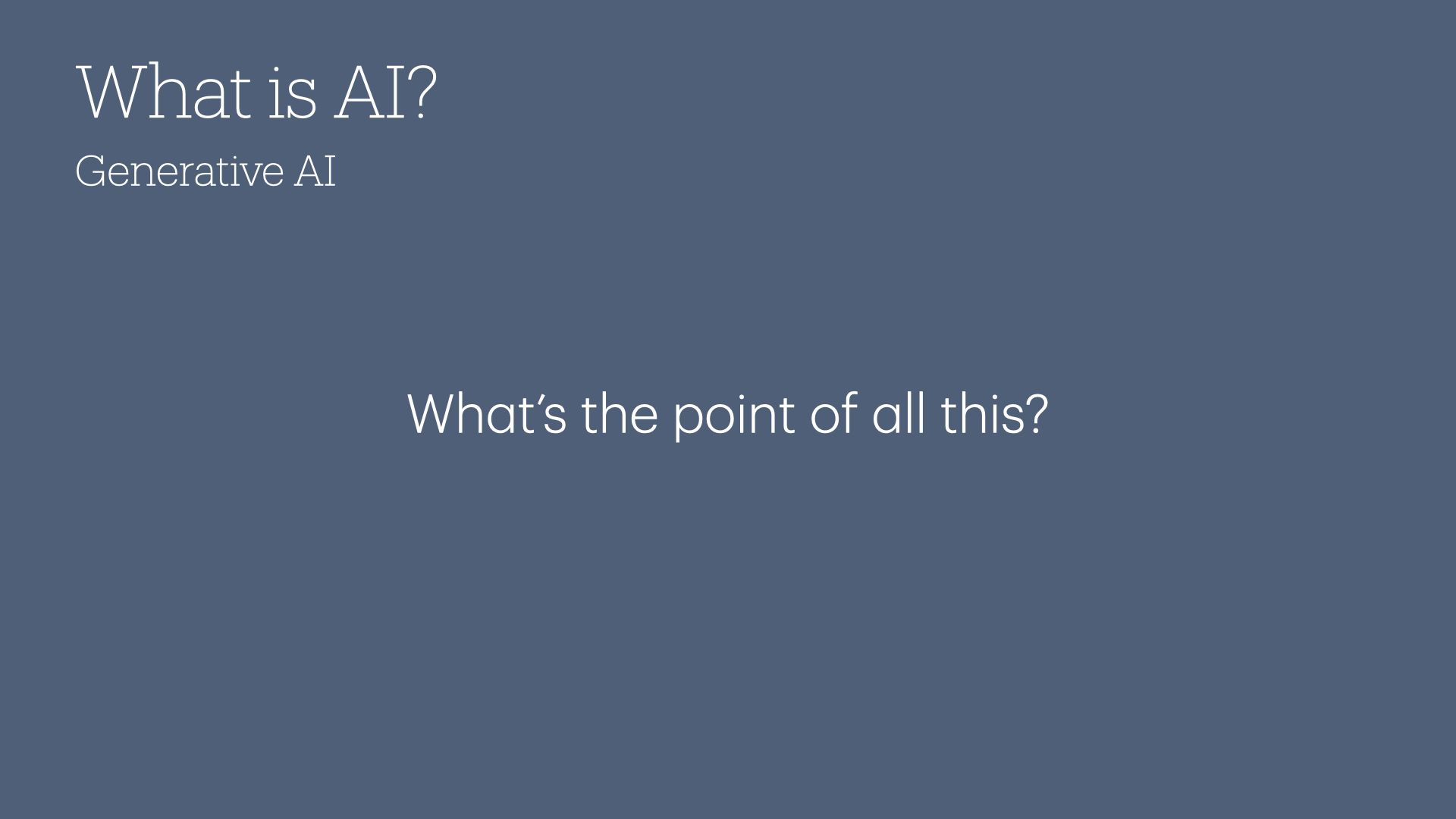
I get that this is a lot, and it's overwhelming to have sixty years of advancements in machine learning thrown at you in about ten minutes.
So let's get to the point of all of this. Why does it matter that we have a computer program that just predicts the most likely word to finish a sentence?
Because it turns out that there are plenty of cases where it's really helpful to get the most likely response to a question!
It's not like you'd want to trust these things implicitly, because as we know, life doesn't always align with what is average.
So when we say "don't trust these things because they're not telling the truth", we mean it! They're not built to be "truthful"; they're built to be "the most likely to be truthful" (which is a big nitpick, for sure, but an important nuance to understand when working with AI!).
Take legal advice, for example. Again, do not trust these things for legal advice, but let's say you need to draft a non-disclosure agreement.
In the old days, you would go to a lawyer who would pull out their own template, make some specific modifications to fit your needs, and pass it along. There's three delicious billable hours right there.
Today, you could go to a large language model and describe the sort of things you'd want your NDA to contain. The LLM would then give you the most likely provisions that are included in NDAs. You could then take that draft and shoot it to your attorney for review. That's 30 billable minutes instead of 3 billable hours.
That's the power of AI. That's why I'm so excited for these generative AI tools. They aren't going to replace humans; they're going to augment them.
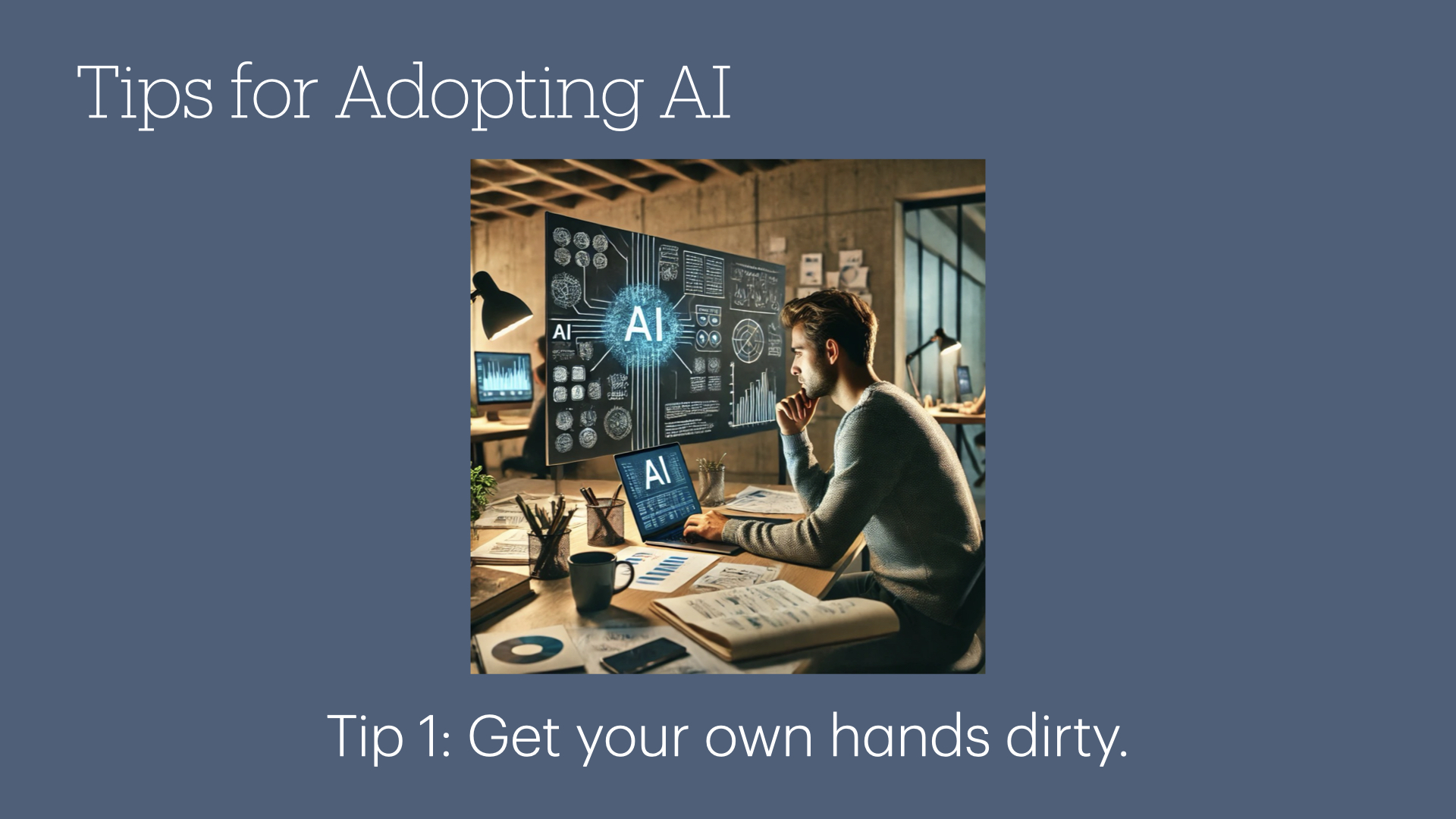
Letâs move on to some practical tips for adopting AI in your organization.
My first tip: you gotta get your own hands dirty and get hands-on experience with these tools.
As a leader, experimenting directly with these tools will help you understand their potential and limitations.
In my career so far, I've noticed that most companies follow a path of hiring consultants to come in and help them adopt new technology. With AI, I encourage you to get familiar with it yourself before shelling out for third party advice.
Action step: Encourage yourself and your employees to use AI tools like ChatGPT for small tasksâdrafting emails, summarizing reports, or answering questionsâand share what they've learned with the team.
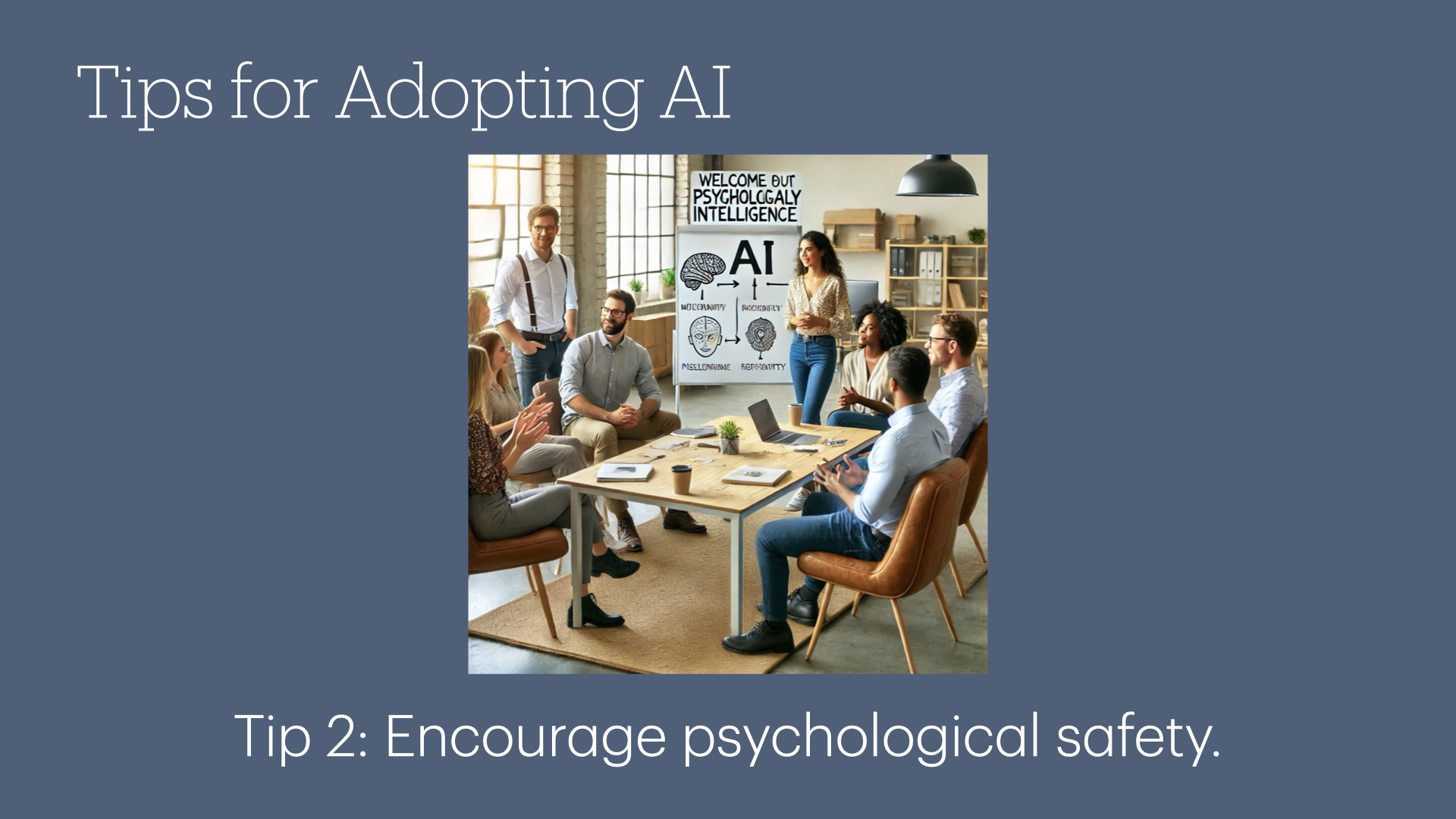
My second tip is to foster psychological safety.
AI adoption requires trial and error, and studies show many employees hesitate to use AI tools at work due to fears of being seen as cheating or potentially automating themselves out of a job.
Create a culture where experimenting with AI is encouraged and celebrated.
Action step: Try running an âAI hackathonâ where employees explore AI tools in a low-stakes environment, share their findings, and foster team learning.
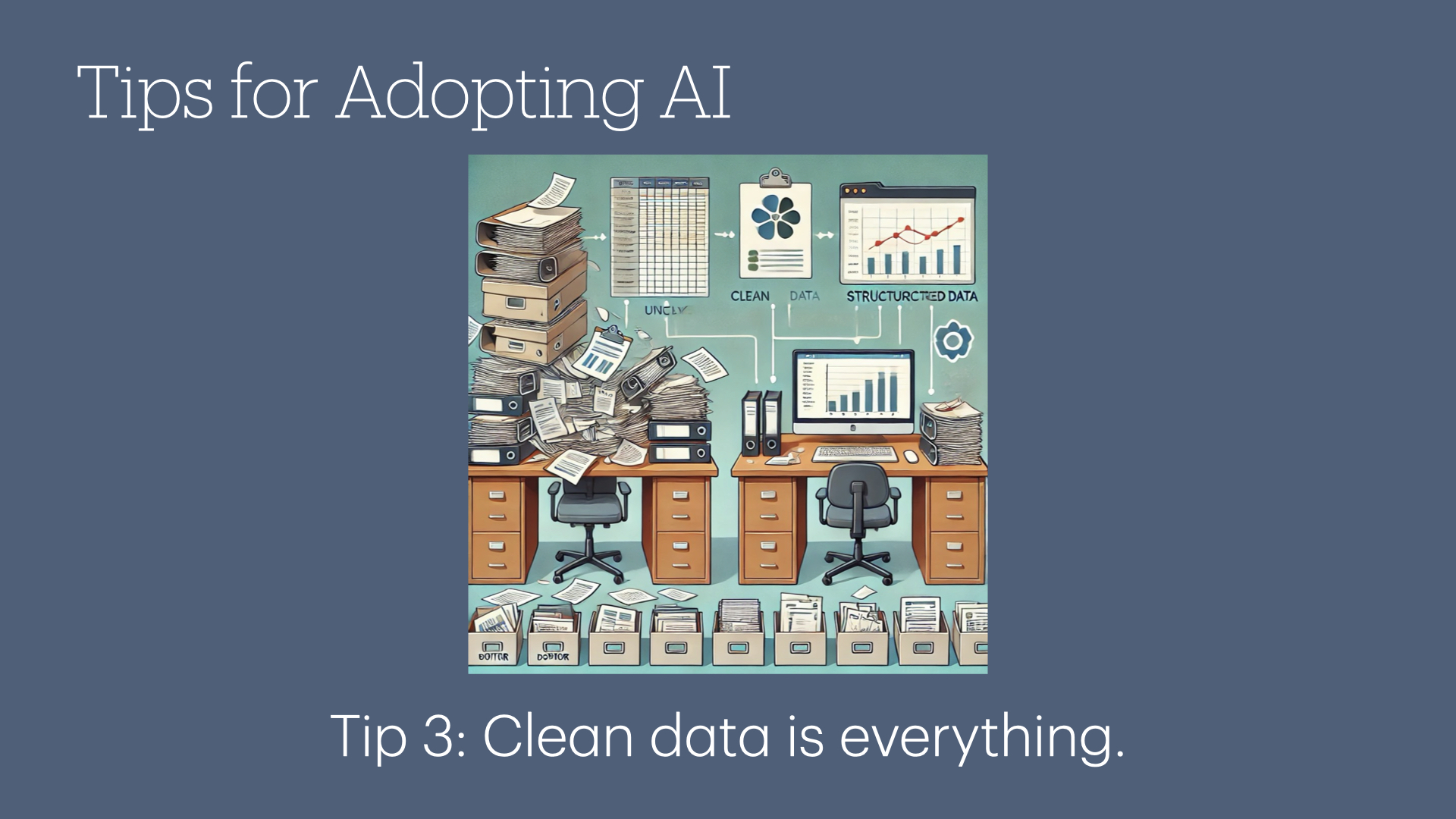
Third: clean data is essential.
AI models are only as good as the data theyâre trained on, so ensure your organizationâs data is organized and free from errors. The better your data, the better your AI models will perform.
And as we'll discuss in the pitfalls section: "dirty" data will lead to biased and inaccurate results.
Action step: Every company has at least one person who loves working with spreadsheets; tap into their skills to spearhead data-cleaning initiatives.
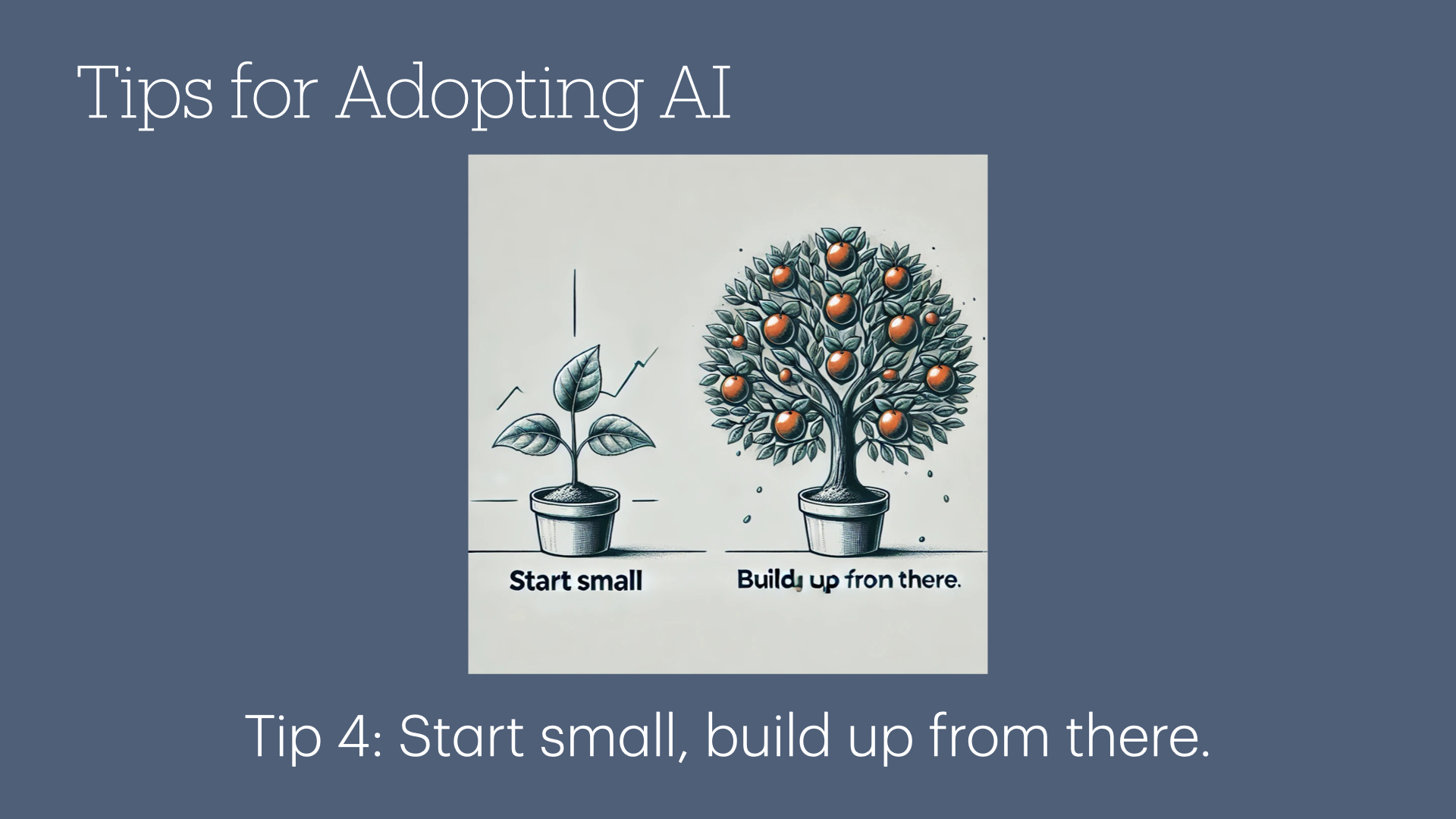
The fourth tip: start small.
Donât try to replace entire workflows with AI right away. Start small, focusing on simple, manageable projects, and scale based on what works.
A great place to start is inviting an AI bot into your virtual meetings to record notes and generate summaries. Be careful to not set it up to "auto join" every meeting (you probably don't want it in a sensitive HR meeting, for example), but give that a try and see how it performs for you.
Action step: Try using AI to do event survey analysis, basic donor segmentation, or create copy for your newsletters or social media channels.
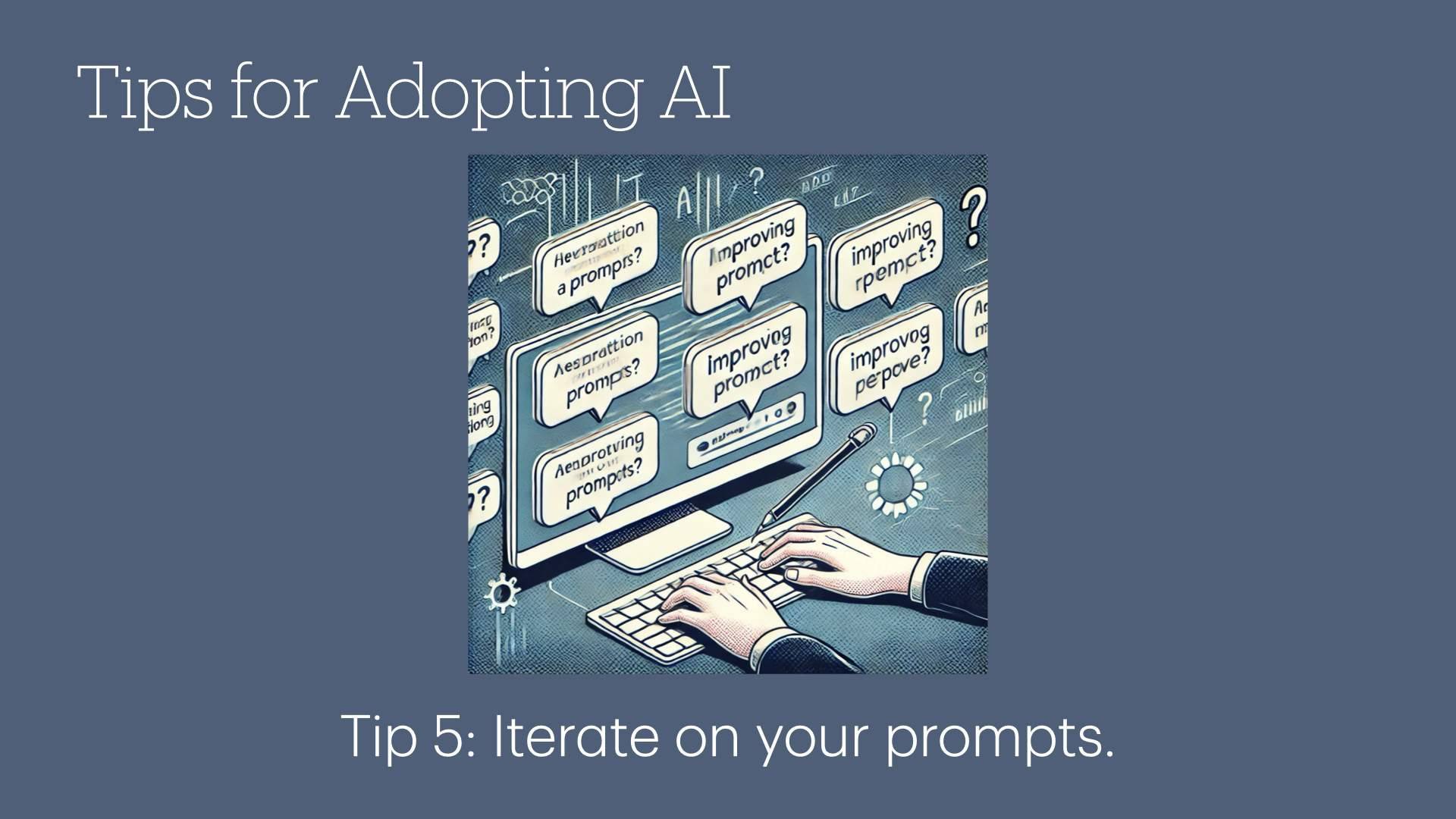
Finally, I can't overstate the importance of continually iterating and improving on your prompts.
Remember our "store/hardware store" example? One word made a world of difference in the output.
Similarly, providing an LLM with a prompt like "Summarize this report" will yield different results from "Create a one-paragraph summary highlighting the most important program outcomes from this report."
The field of research which tries to figure out how to get the most out of these tools is called "prompt engineering". You can find tons of great resources online and on YouTube for how to best phrase things for different types of models. For example, the prompts that work best for ChatGPT are different than Claude. And the prompts you use for a text generator will be different than an image generator like Midjourney.
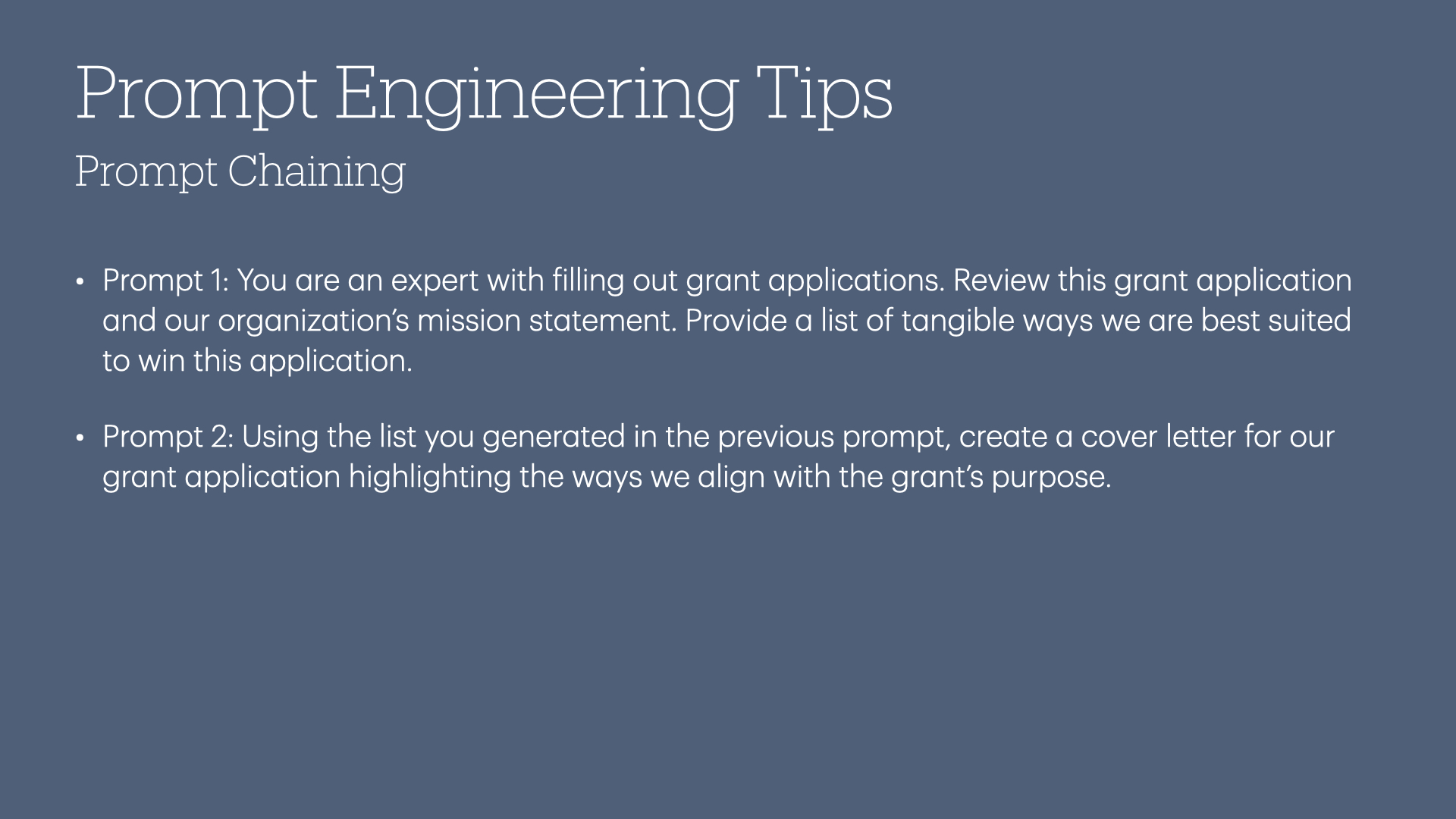
A prompt engineering tricks that I use all the time is called "prompt chaining."
Prompt chaining involves using the result from one prompt as the foundation for the next prompt.
Instead of asking an LLM to generate a cover letter for a grant application, you could first ask an LLM to review both a grant application and your organization's mission, and then provide a list of areas where there are synergies.
Then, you can take the results from that and ask it to write the letter.
Giving the models time to reason through their answers tends to lead to better outcomes.
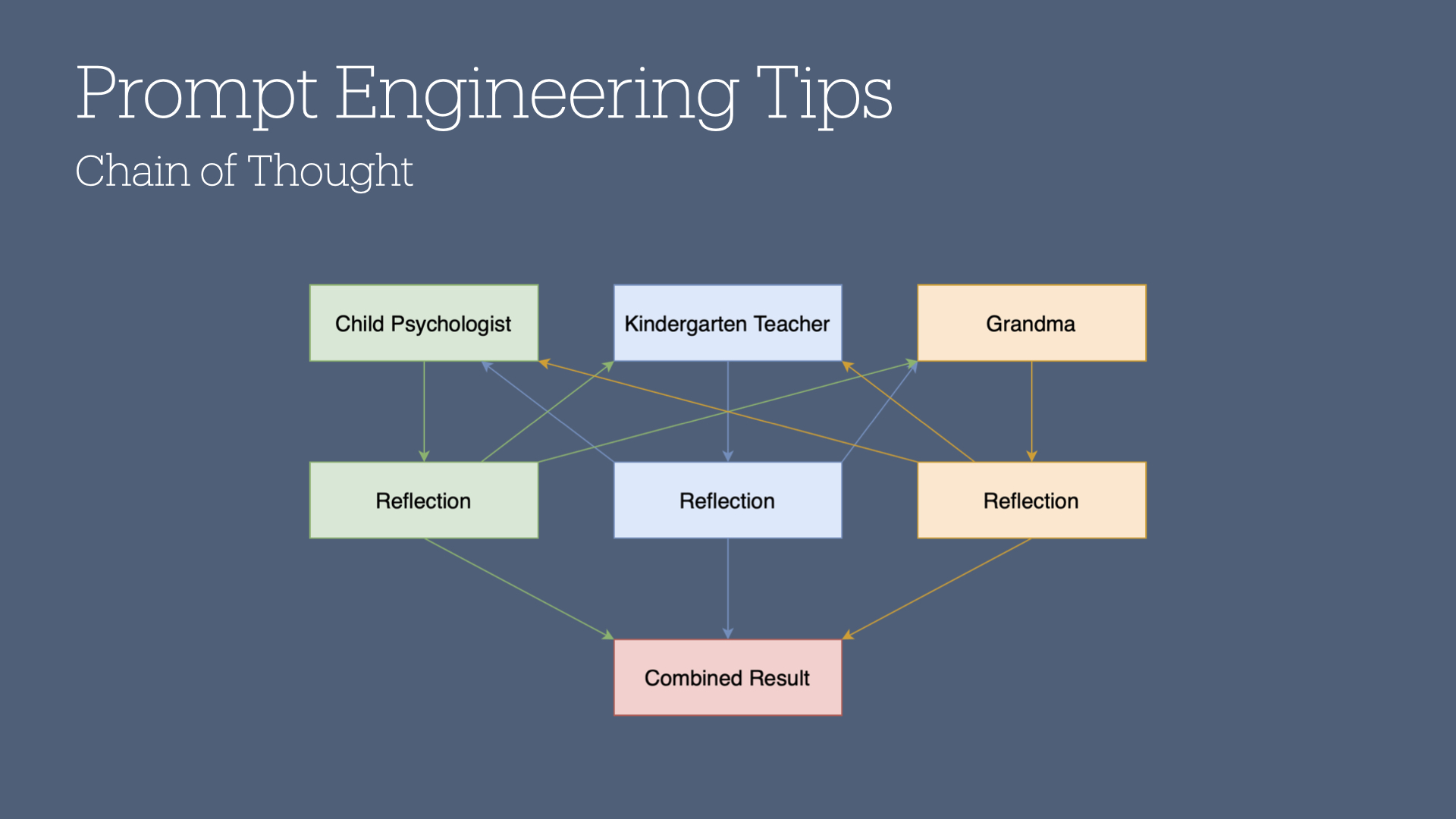
Another prompt engineering trick I frequently reach for is called chain of thought.
With this technique, you are asking an LLM to think about a given problem from three distinct perspectives. You then ask it to act as one of those personas and critique the responses of the other two. Finally, you combine the results into a well-considered and well-rounded answer.
As an example: my son does not like to eat pizza. I know... it bums me out, too.
I provided ChatGPT with a bunch of backstory on my son and what we've tried to do to encourage him to try pizza. Then, I said to pretend you are a kindergarten teacher, a child psychologist, and a grandparent. As each of those personas, tell me what approach you would take to get my son to eat pizza.
Next, as each persona, I ask it to reflect on the answers of the other personas. For example, the child psychologist persona would consider the kindergarten teacher and grandma's perspectives and adjust their own response.
Finally, after all personas have reflected on each other's answers, I have the model summarize the best path forward.
This trick works exceptionally well across several different problems. As an engineer, I use it to consider system changes as an engineer, as an end user, and as a business executive. It can provide some insights which you may have otherwise missed.
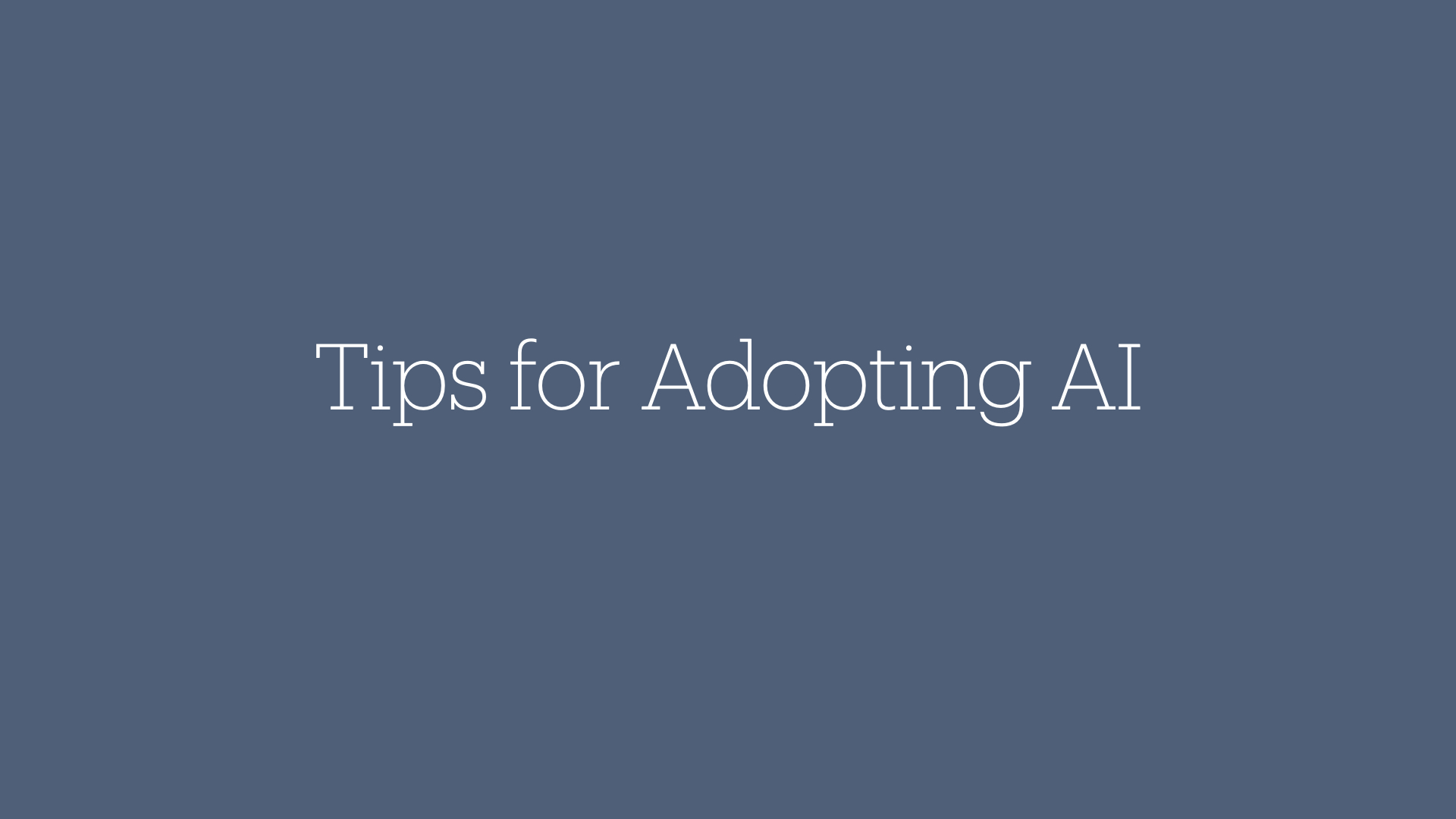
So in order to integrate AI successfully, treat it as a tool that augments, rather than replaces, human judgment.
Every time I fire up an AI assistant, I like to think of it as an eager intern who is exceptionally smart but exceptionally naive. I do not take its output as gospel; rather, I use it as a foundation and build on it from there.
The best way to integrate AI into your workflows is to use it for routine tasks, and keep human oversight for critical decisions.
Finally, I'll take this time to further emphasize that all AI outputs are based on probability, not the truth. Always review and adjust outputs as needed.
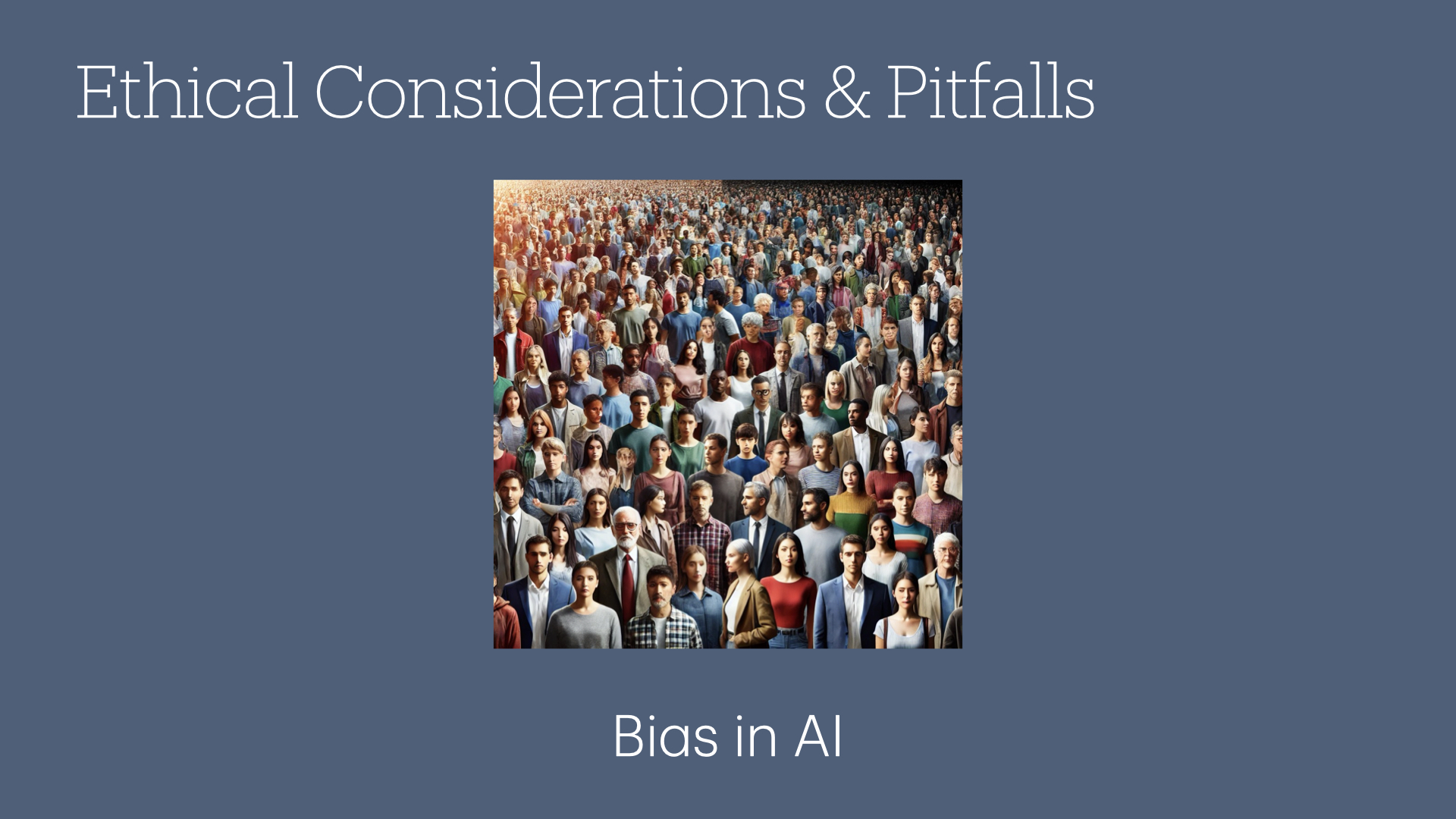
Alright, we've covered what artificial intelligence is, and we've gotten through some tips for adopting AI into your organization.
Now, let's talk about areas where AI can fall flat.
First: bias.
If you recall, at the beginning of this talk, we described artificial intelligence as being focused on getting computers to be like humans.
Humans are inherently biased, and AI, trained on human-generated data, often reflects this bias. Achieving true âunbiasedâ AI is a complex, if not impossible, task.
I propose you think of AI in the same context: there is no such thing as an unbiased AI model.
AI models are only as good as the data with which you train it. Data is one of those things you can pretty easily screw up if you aren't attuned to all of the various forms of bias that could impact your data.
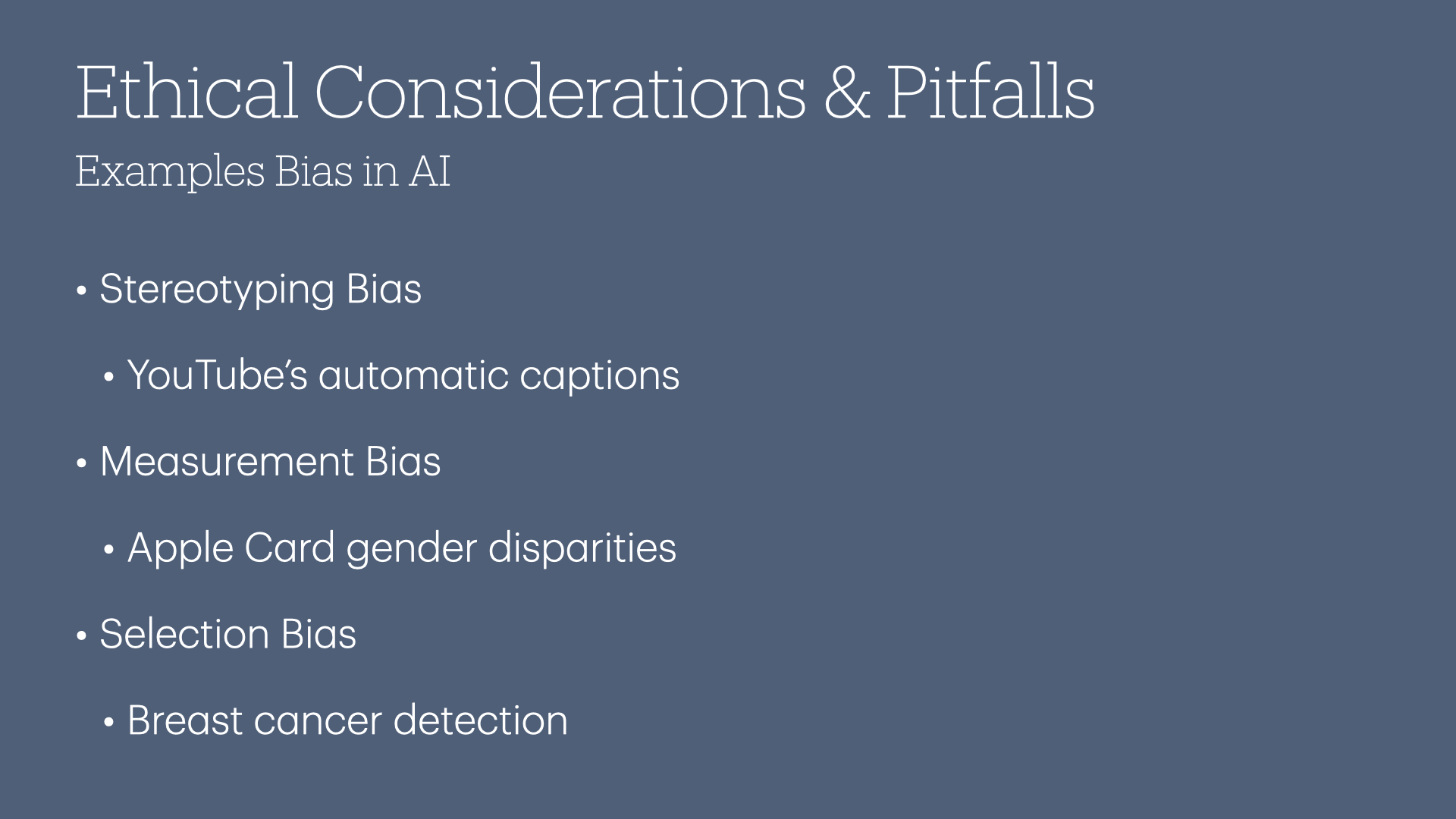
There are many different kinds of bias, but I wanted to highlight three specific forms as a starter:
Stereotyping bias: This occurs when AI models perform less accurately for certain groups due to their underrepresentation or misrepresentation in training data, as seen with YouTube's automatic captions, which struggle with Scottish, Indian, and African American accents.
Measurement bias Measurement bias happens when an AI modelâs metrics or algorithms lead to systematically skewed outcomes, such as the Apple Cardâs algorithm offering men higher credit limits than women with similar financial profiles.
Selection bias: Selection bias arises when training data lacks sufficient diversity, causing models to underperform for certain groups; for instance, breast cancer detection AI trained mainly on female patients performs less accurately for male patients.
There are many more forms of bias that you can research on your own, but the main takeaway here is that all systems are subject to bias depending on what data was used to train it. For this reason, you can't just rely on the output of an AI-led decision.
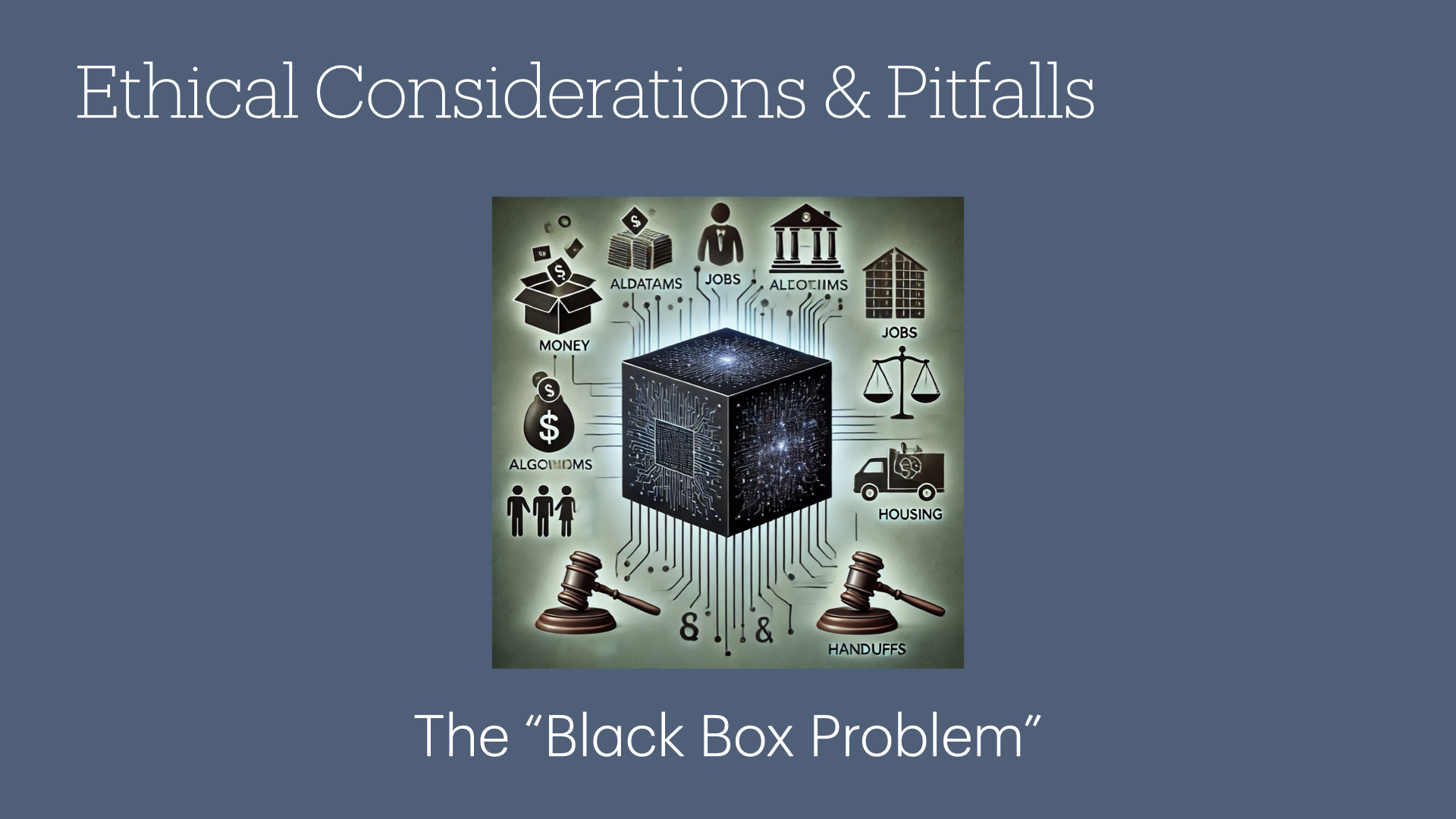
As mentioned earlier, another major issue is the âblack boxâ problem.
Deep learning models are like locked safesâeach layer hides its âreasoningâ behind many interconnected processes, making it nearly impossible for humans to interpret every decision-making step.
This lack of transparency, especially in high-stakes areas like criminal justice or credit scoring, means weâre left trusting the âsafeâ without ever seeing inside, creating ethical and practical risks.
Once again, this is a reminder that we canât just accept AI output as absolute truth; careful consideration and oversight are needed to avoid unintentional discrimination or bias.
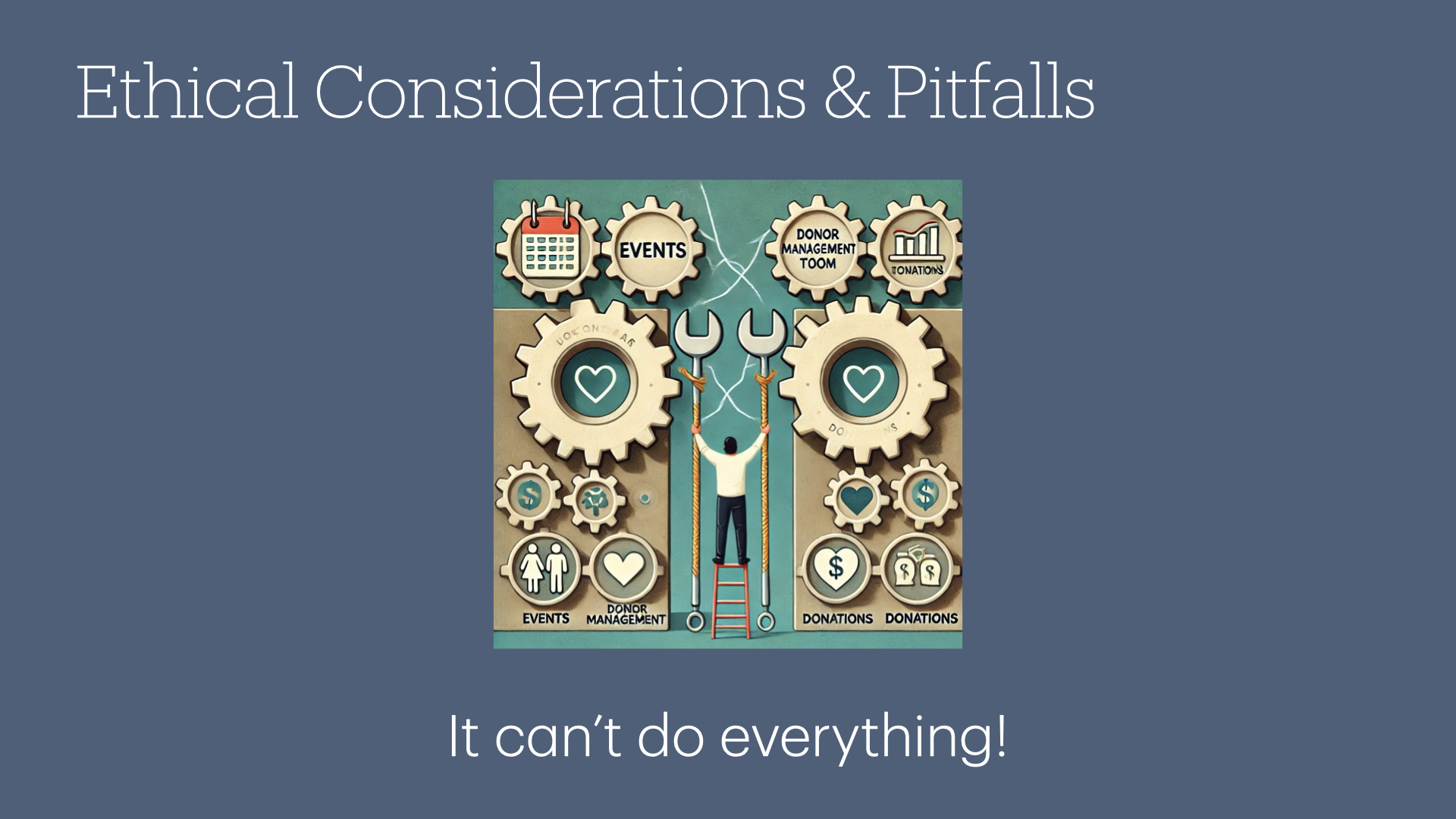
Literally every single time new technology drops, some wise guy emerges from the crowd and says, "well, I can't use [insert new tech] to do [insert obvious use case]".
Earlier in this talk, I led off by saying "AI is for everyone." Notice how I didn't say "AI is for every thing."
Of course you can't use AI for everything! AI is not a magic bullet. You gotta know how to deploy it effectively, which is in service of automating predictable, repetitive tasks.
Yes, wise guy, you are right: you aren't gonna want to deploy AI while leading a camping expedition in the Boundary Waters.
But after you complete your expedition and ask for feedback from the program's participants, you could use AI to process those responses and bucket them into understandable and actionable groups.
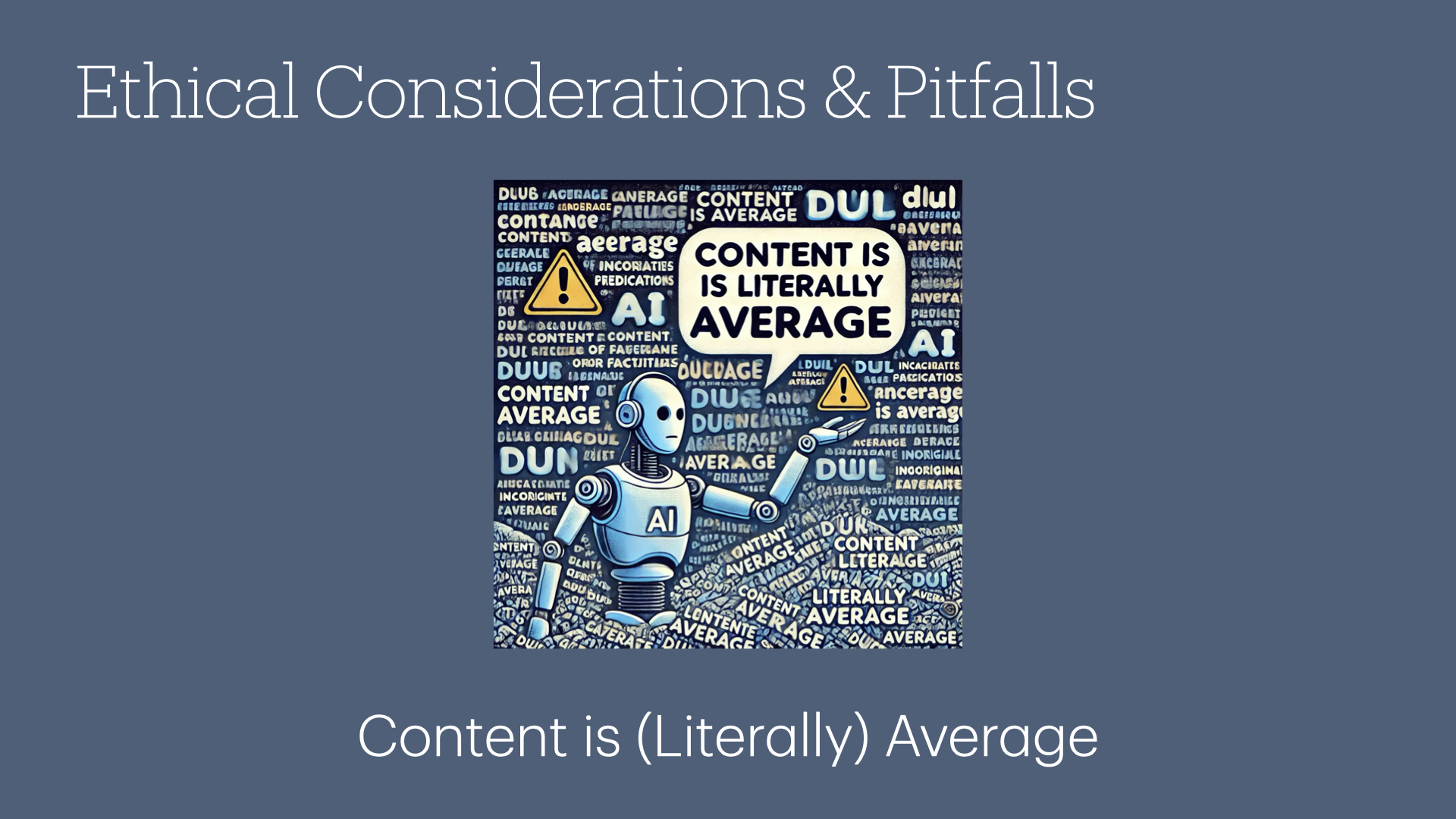
If you've been paying attention during this entire talk, you'll notice I keep saying things like "AI is picking the most likely word to finish a sentence" and "machine learning is used to make predictions."
If you are relying on a tool to create the most likely response to something, you'll see quickly that the responses are kinda... average.
This can be advantageous, but it's also something to be aware of. By using output that is average by design, you run the risk of blending into everything else out there. (This, by the way, leads to the rise of slop, which is the AI equivalent of spam).
Now, this may be a trade off you are willing to accept in many cases. I, for one, often use AI as a therapist to help me make sense of some thoughts swirling around in my head. This works great, but I use the advice and feedback I get from the model and take it to a human therapist.
The other thing about the content being average: remember how we said that AI doesn't care about truthiness, but rather it cares about finding the thing that is most likely to be truthful? This leads to some concerning behavior called "hallucination", where it will make up facts which aren't actually facts.
You may recall headlines from a year ago where a lawyer used ChatGPT and it hallucinated cases. This sort of thing happens all the time with new technology, especially when it's used by people who aren't properly trained on how to use it (or are swayed by glitzy marketing campaigns which make promises that it can't possibly deliver).
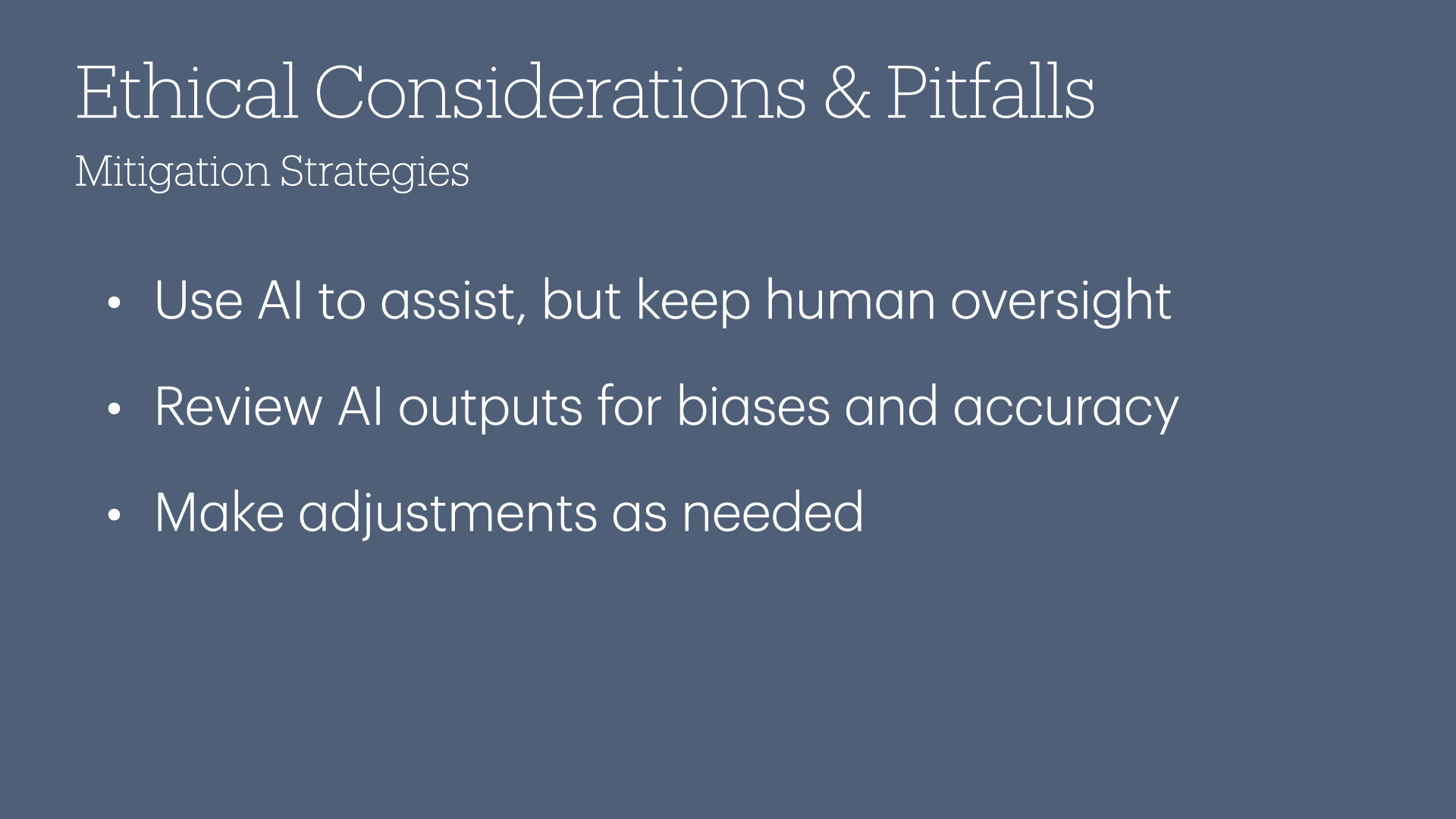
Now that you're aware of the pitfalls and risks of using artificial intelligence, how can you mitigate those risks?
Always treat AI as a supportive tool, maintaining human oversightâespecially for important decisions where ethics and accuracy are critical.
Always review AI outputs for potential bias and inaccuracies.
Finally, adjust AI-generated content as needed to match your style and objectives. For instance, AI may draft a social media post, but tweaking it to align with your brand's voice adds value.
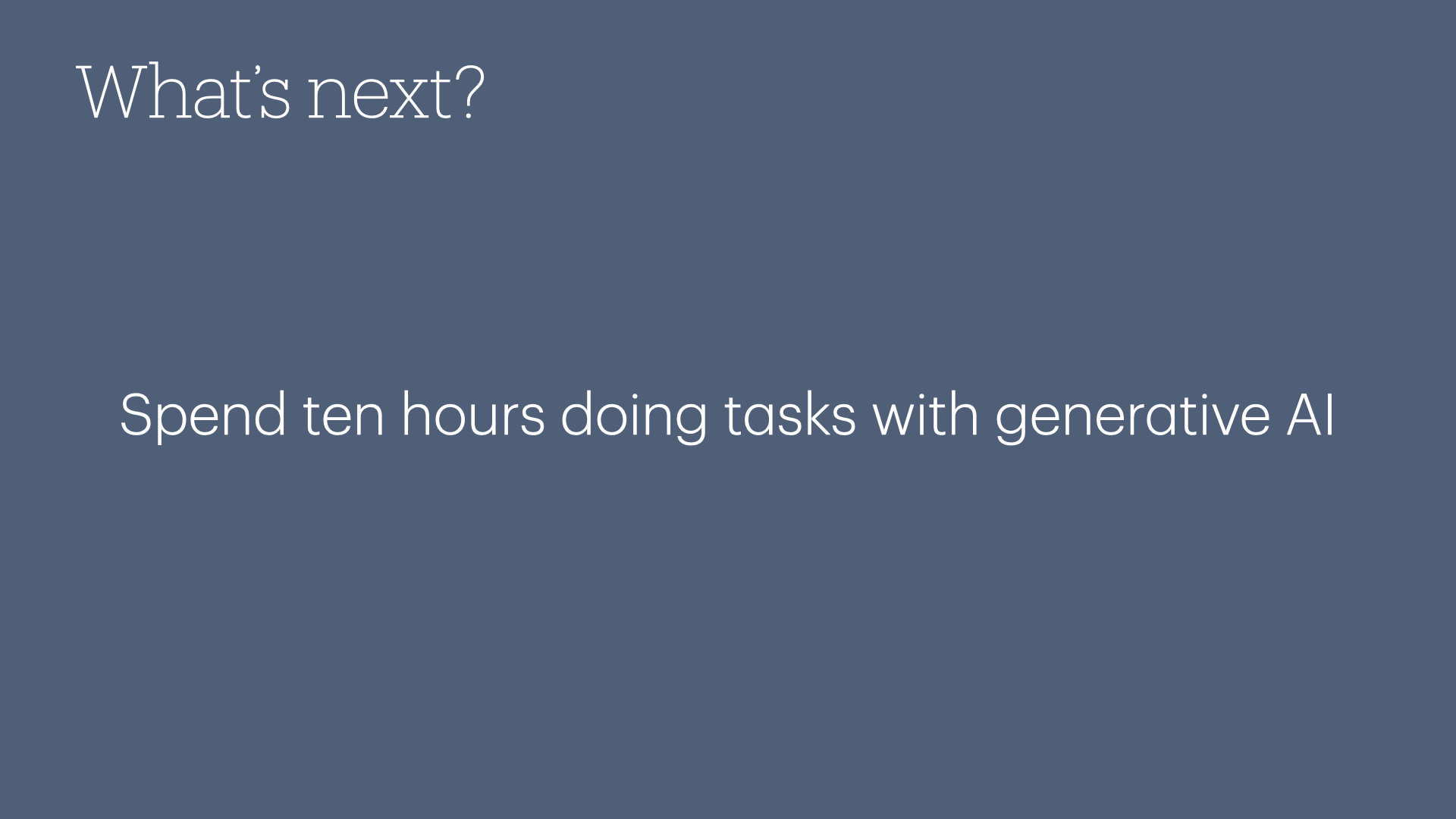
We've covered what AI is, practical tips for adopting it, ethical concerns, and common pitfalls.
So, what's next for you?
Begin by dedicating 10 hours to using generative AI tools to build practical familiarity.
Try asking questions in areas you know well to see how AI performs, and notice where youâd add or change things.
Sharing what you learn with your team encourages experimentation and fosters a learning environment.